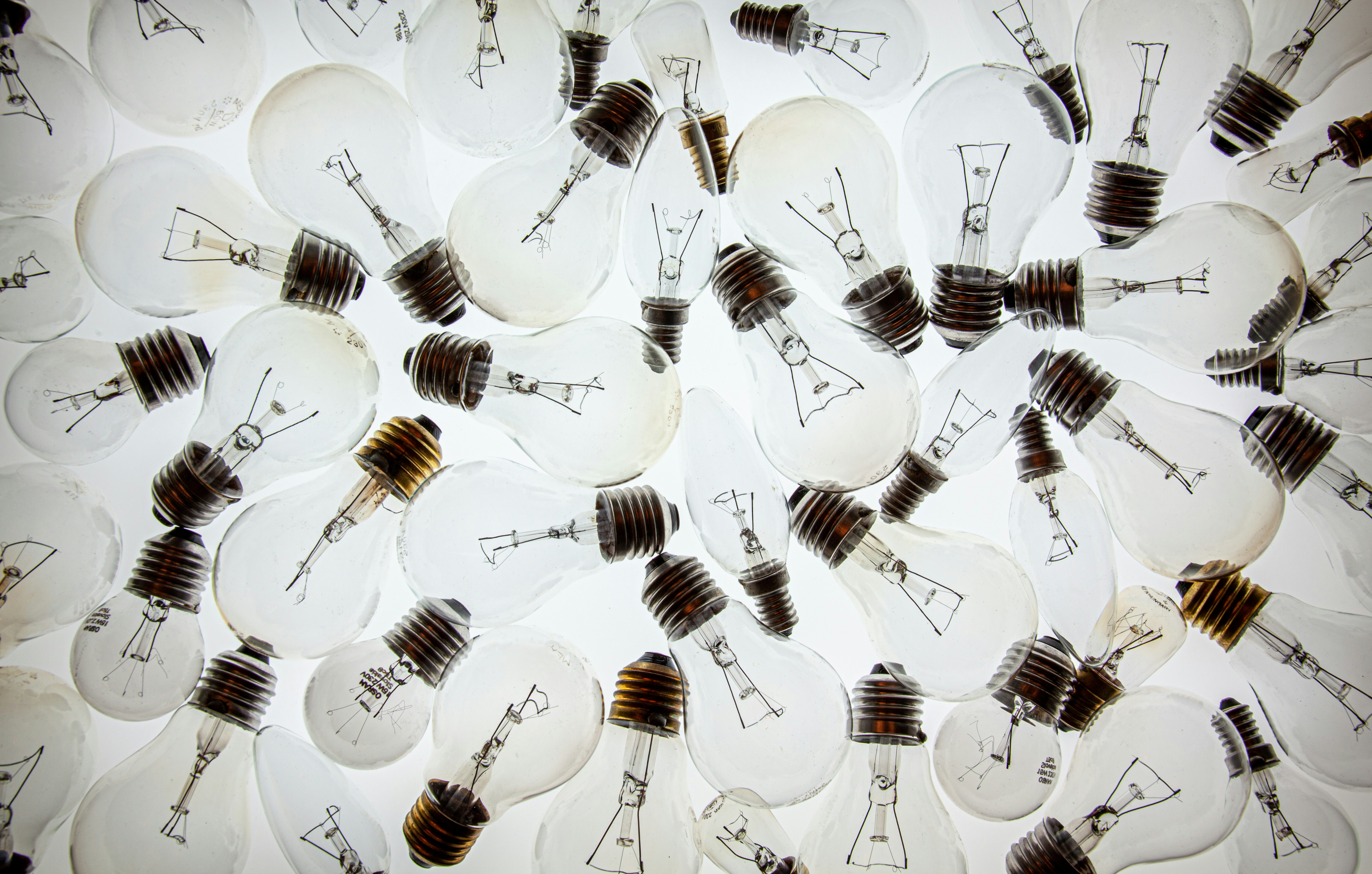
Tips for Staying Inspired: How Machine Learning Pros Fuel Creativity and Innovation
Machine learning (ML) continues to reshape industries—from personalised e-commerce recommendations and autonomous vehicles to advanced healthcare diagnostics and predictive maintenance in manufacturing. Yet behind every revolutionary model lies a challenging and sometimes repetitive process: data cleaning, hyperparameter tuning, infrastructure management, stakeholder communications, and constant performance monitoring. It’s no wonder many ML professionals can experience creative fatigue or get stuck in the daily grind. So, how do machine learning experts keep their spark alive and continually generate fresh ideas? Below, you’ll find ten actionable strategies that successful ML engineers, data scientists, and research scientists use to stay innovative and push boundaries. Whether you’re an experienced practitioner or just breaking into the field, these tips can help you fuel creativity and discover new angles for solving complex problems.