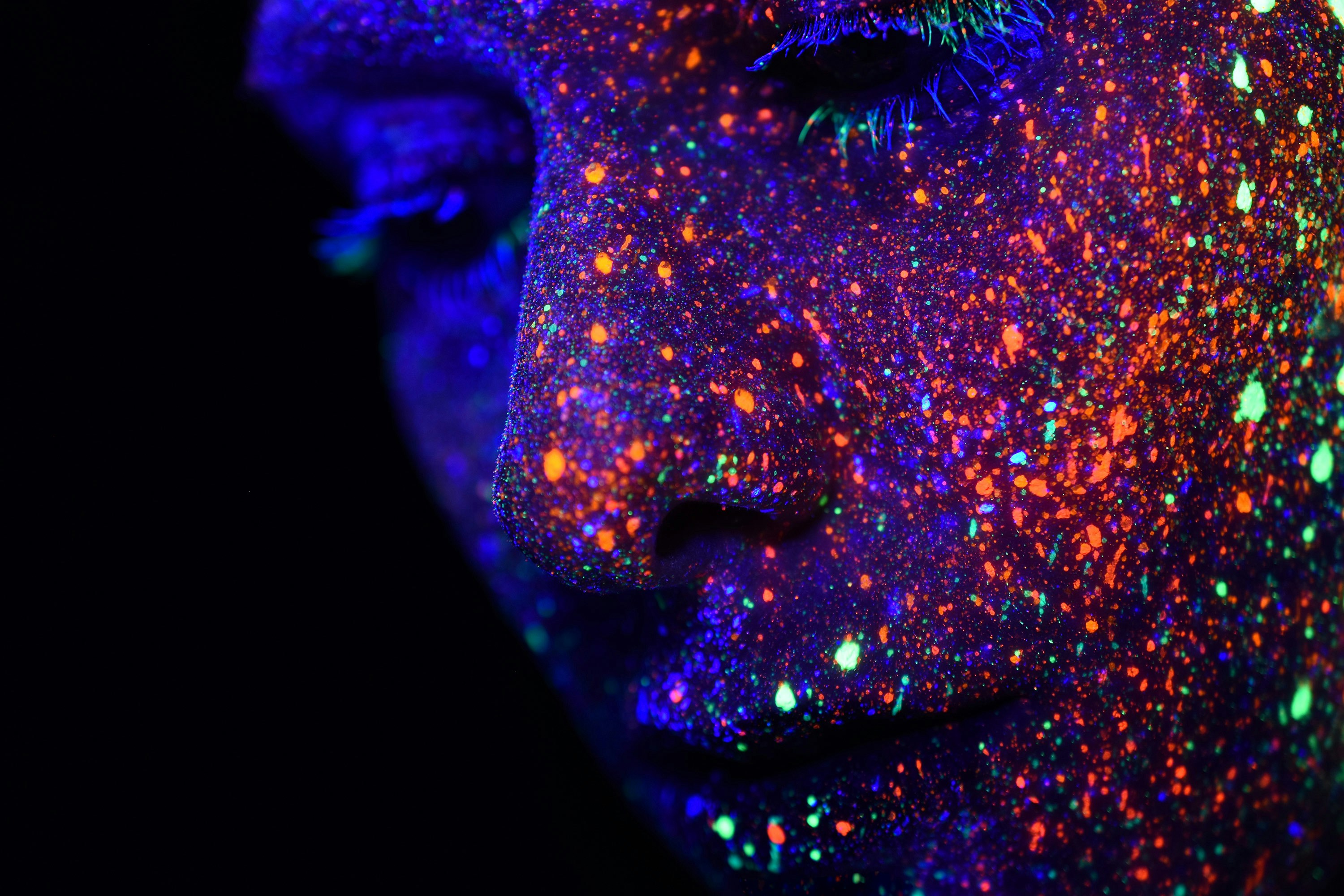
Key Trends Shaping Machine Learning Jobs in the UK in 2025
In today’s rapidly evolving technology landscape, machine learning (ML) has emerged as one of the most sought-after skill sets in the UK job market. From healthcare and financial services to retail and autonomous systems, ML professionals are in high demand across sectors. However, as the industry continues to advance, job seekers must stay aware of the key trends shaping the future of machine learning roles.
In this article, we’ll explore the most significant trends likely to influence hiring in the ML sector over the next 12 months. We’ll cover topics such as low-code and no-code ML tools, hybrid AI models, and AI regulation, offering insight into what these changes mean for aspiring and experienced ML professionals.
1. The Rise of Low-Code and No-Code Machine Learning
What is Low-Code and No-Code ML?
Low-code and no-code platforms are revolutionising the ML space by enabling users with little to no programming experience to build and deploy machine learning models. Platforms such as Google AutoML, Microsoft Azure ML, and DataRobot allow users to automate the most technical aspects of the ML pipeline—like feature engineering and model selection—through intuitive drag-and-drop interfaces.
Why Does This Matter for ML Jobs?
Low-code and no-code tools are democratising access to machine learning, allowing businesses to integrate AI capabilities without needing a full team of ML engineers. However, this trend does not replace skilled professionals—it redefines their role.
Shift in Focus: ML engineers will move away from building models from scratch and focus more on optimising models, managing complex datasets, and ensuring responsible AI deployment.
Upskilling Needs: ML professionals who understand how to use these tools effectively will stand out. Knowledge of platforms like DataRobot, KNIME, and Azure ML will be in high demand alongside traditional skills such as Python and TensorFlow.
Business Collaboration: Non-technical teams such as marketing, HR, or operations will increasingly use low-code ML tools. ML professionals will be required to bridge the gap between these teams and the models being implemented.
Takeaway for Job Seekers:
Adaptability is key. Job seekers should embrace low-code tools to complement their coding skills. Focus on combining these platforms with a deeper understanding of model optimisation, interpretability, and deployment to deliver value to employers.
2. Hybrid AI Models: Combining Classical and Deep Learning Approaches
What Are Hybrid AI Models?
Hybrid AI models combine traditional machine learning techniques (like decision trees and logistic regression) with modern deep learning approaches. These models aim to maximise the strengths of both approaches, such as interpretability and computational efficiency on the classical side, and the ability to handle unstructured data on the deep learning side.
For example, in financial services, hybrid models are being used to combine structured transaction data with unstructured text or image data for fraud detection.
How Hybrid Models Will Impact Hiring
The integration of hybrid models will create opportunities for job seekers with a diverse set of skills. Employers will look for professionals who can:
Build Multimodal Systems: Combine text, image, and numerical data into a unified ML pipeline.
Optimise Complex Workflows: Understand when to apply classical ML methods versus deep learning frameworks to improve accuracy and efficiency.
Solve Real-World Problems: Hybrid models are often more practical for solving business-specific challenges, such as predictive maintenance, demand forecasting, or personalised recommendations.
Takeaway for Job Seekers:
To stay ahead, job seekers should expand their skill set to include both classical and deep learning techniques. Developing expertise in tools like scikit-learn (for classical ML) and PyTorch or TensorFlow (for deep learning) will be a major asset. Additionally, understanding frameworks for multimodal models, such as Hugging Face’s Transformers, will provide a competitive edge.
3. Growing Focus on AI Regulation and Ethical AI
Why AI Regulation is Increasing
With the UK government placing a greater emphasis on AI governance and ethical use, AI regulation is becoming a priority. This includes ensuring data privacy, addressing algorithmic bias, and improving the transparency of AI systems. For example, the UK’s AI White Paper outlines regulatory guidelines to promote innovation while safeguarding public trust.
As AI regulation becomes more robust, organisations will need machine learning professionals who can build compliant, ethical, and transparent systems.
Impact on Machine Learning Jobs
Regulation will drive demand for the following skills:
Explainable AI (XAI): Employers are looking for professionals who can build transparent ML models where decisions are interpretable. Knowledge of techniques like SHAP (Shapley Additive Explanations) and LIME (Local Interpretable Model-Agnostic Explanations) is essential.
Bias Detection and Mitigation: ML professionals must know how to assess and mitigate bias in datasets and models, ensuring fairness and inclusivity.
AI Governance: Businesses will need professionals who can align AI systems with regulatory requirements. Roles such as AI Governance Specialists or Ethical AI Consultants are emerging.
Takeaway for Job Seekers:
Familiarise yourself with ethical AI principles, regulatory frameworks, and explainable AI techniques. Adding certifications such as AI Ethics by the World Economic Forum or AI & Law by FutureLearn can demonstrate your awareness and understanding of these critical trends.
4. Machine Learning in Edge Computing
What is Edge Computing?
Edge computing involves processing data closer to its source (e.g., on IoT devices or edge servers) rather than sending it to a centralised cloud infrastructure. This reduces latency, improves real-time performance, and conserves bandwidth.
Machine learning on the edge is gaining momentum in sectors such as autonomous vehicles, smart devices, and industrial IoT systems.
How It Will Impact Hiring
The growth of edge computing will lead to demand for machine learning engineers who can:
Optimise Models for Edge Deployment: Knowledge of model compression, quantisation, and frameworks like TensorFlow Lite or ONNX is increasingly important.
Integrate ML with IoT: Employers will seek professionals who can combine machine learning expertise with IoT protocols like MQTT and edge platforms such as AWS Greengrass.
Manage Limited Resources: Building ML solutions for edge devices involves dealing with constraints like limited computational power and energy efficiency.
Takeaway for Job Seekers:
Develop skills in lightweight model development, hardware-aware optimisation, and IoT integration. Understanding frameworks like TensorFlow Lite, OpenVINO, or Edge TPU will be advantageous.
5. The Demand for Multidisciplinary ML Professionals
Why Multidisciplinary Skills Matter
As ML adoption increases, professionals are expected to understand the broader business and domain context. For example:
Healthcare: ML engineers working on medical imaging need to understand clinical workflows and regulatory considerations.
Finance: ML professionals are required to develop fraud detection algorithms while adhering to strict compliance laws.
Retail: Building recommendation engines requires both technical expertise and a solid grasp of customer experience.
Emerging Roles:
The trend towards multidisciplinary work is creating hybrid roles such as:
AI Product Managers: Professionals who can align ML solutions with business goals and customer needs.
AI Translators: Individuals who act as liaisons between technical teams and non-technical stakeholders.
Domain-Specific ML Engineers: Experts who combine ML knowledge with niche industries, such as healthcare, law, or insurance.
Takeaway for Job Seekers:
To stand out, build expertise in a specific industry alongside your ML knowledge. For instance, if you aim to work in healthcare, consider learning about medical terminologies, patient data privacy regulations, and healthcare AI use cases.
6. Automation in MLOps and Model Deployment
What is MLOps?
MLOps (Machine Learning Operations) is the practice of automating and managing the ML lifecycle, from data preparation and model training to deployment and monitoring. As businesses scale their ML initiatives, MLOps is becoming a critical part of operations.
The Growing Need for Automation
The increasing adoption of automated tools for CI/CD pipelines, model retraining, and monitoring means ML professionals must be proficient in MLOps platforms like:
AWS SageMaker: For end-to-end ML workflows.
Kubeflow: For managing Kubernetes-based ML pipelines.
MLflow: For tracking experiments and deployments.
Impact on ML Jobs:
Professionals with skills in MLOps will be highly valued as companies move towards automating the ML lifecycle.
The ability to deploy models efficiently and monitor their performance in production environments will be a core requirement.
Takeaway for Job Seekers:
Learn MLOps tools and frameworks to differentiate yourself. Proficiency in DevOps concepts (such as Docker, Kubernetes, and Git) combined with ML expertise will make you a standout candidate.
Final Thoughts: Staying Competitive in a Changing Landscape
The machine learning job market in the UK is evolving at a rapid pace, driven by trends like low-code tools, hybrid models, ethical AI regulation, and edge computing. As these trends reshape the skills employers seek, job seekers must adapt to remain competitive.
Here are some actionable steps to future-proof your career:
Embrace Low-Code Platforms: Master tools like Google AutoML or Azure ML while honing your traditional coding skills.
Expand Your Skill Set: Combine classical and deep learning approaches to build hybrid models.
Focus on Ethical AI: Learn explainability, fairness, and governance techniques.
Learn Edge ML: Gain expertise in deploying machine learning models on edge devices.
Develop Domain Expertise: Combine ML knowledge with sector-specific skills.
Adopt MLOps Tools: Gain hands-on experience with automated deployment pipelines.
By staying informed and upskilling in these areas, ML professionals can take advantage of emerging opportunities and thrive in the UK’s dynamic technology job market.
Whether you’re a new graduate or a seasoned machine learning engineer, these trends present exciting pathways for growth, innovation, and career advancement. If you’re ready to explore opportunities, check out the latest ML roles on www.machinelearningjobs.co.uk.