Related Jobs
View all jobsSenior Applied Scientist, Amazon Audiences - ADSP
Data Scientist
Applied Science Manager , Personalization Team
Solutions Architect [Role Based In Abu Dhabi, UAE]
Principal Bioinformatician
Get the latest insights and jobs direct. Sign up for our newsletter.
Industry Insights
Discover insightful articles, industry insights, expert tips, and curated resources.
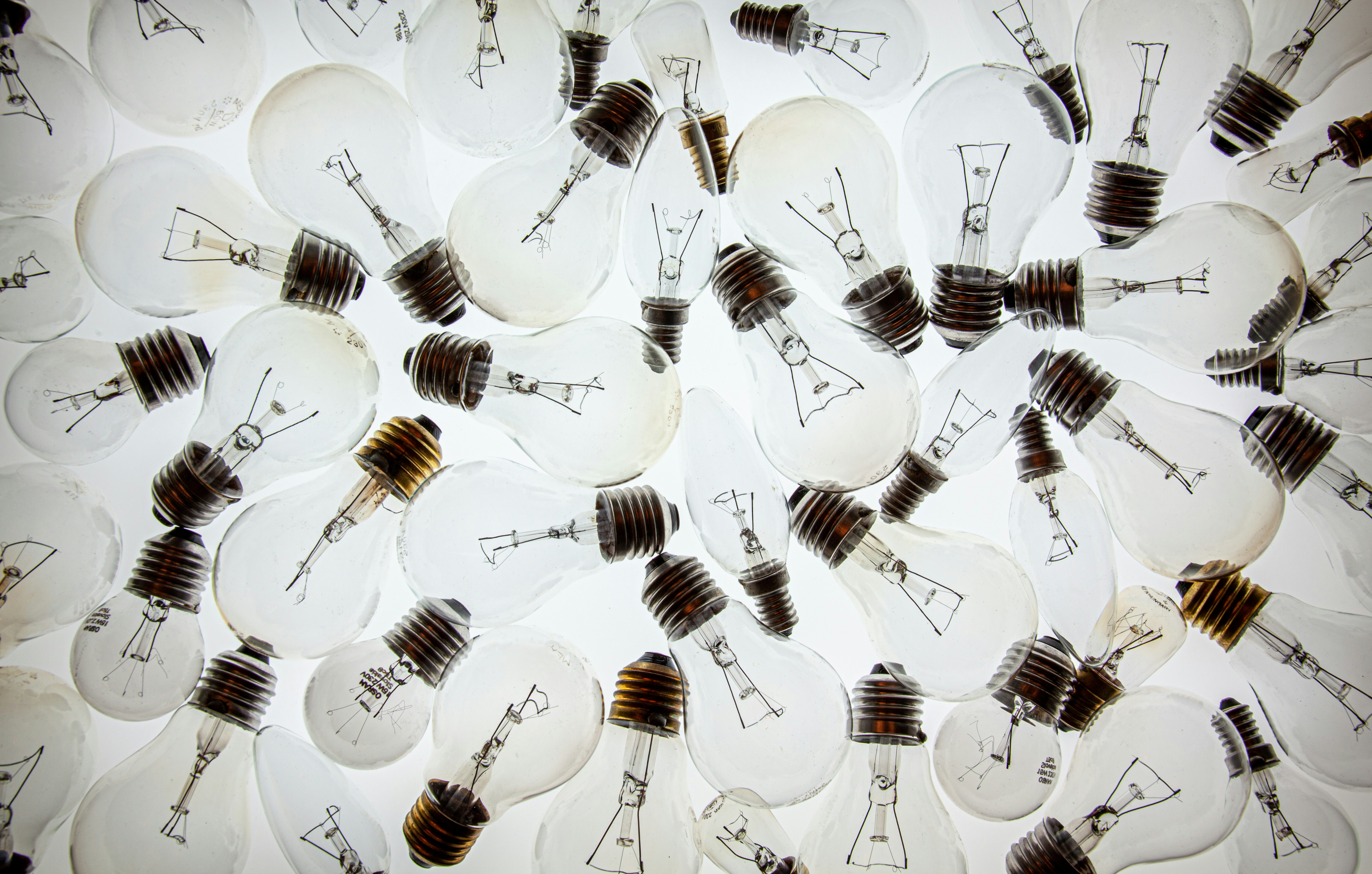
Tips for Staying Inspired: How Machine Learning Pros Fuel Creativity and Innovation
Machine learning (ML) continues to reshape industries—from personalised e-commerce recommendations and autonomous vehicles to advanced healthcare diagnostics and predictive maintenance in manufacturing. Yet behind every revolutionary model lies a challenging and sometimes repetitive process: data cleaning, hyperparameter tuning, infrastructure management, stakeholder communications, and constant performance monitoring. It’s no wonder many ML professionals can experience creative fatigue or get stuck in the daily grind. So, how do machine learning experts keep their spark alive and continually generate fresh ideas? Below, you’ll find ten actionable strategies that successful ML engineers, data scientists, and research scientists use to stay innovative and push boundaries. Whether you’re an experienced practitioner or just breaking into the field, these tips can help you fuel creativity and discover new angles for solving complex problems.
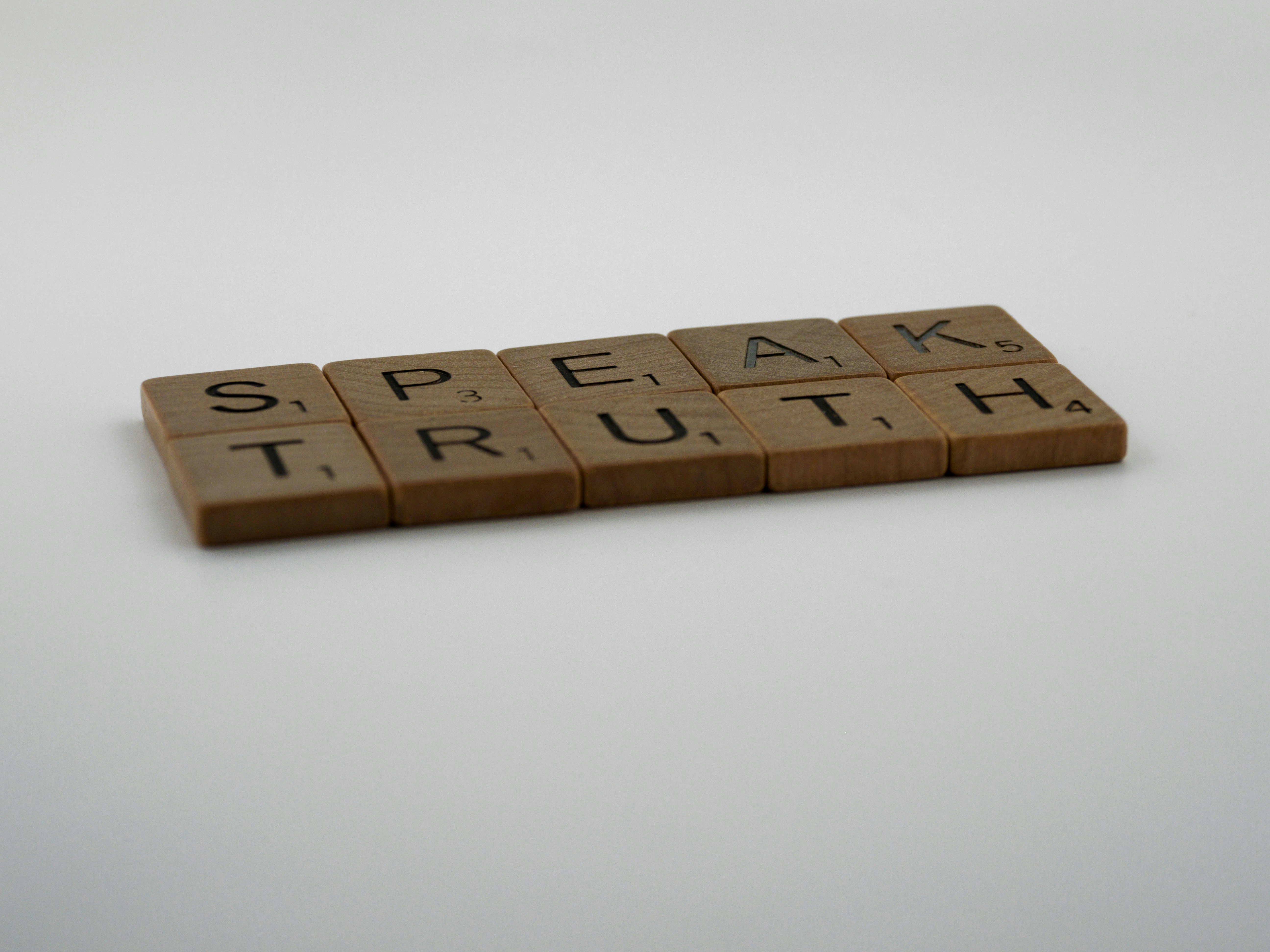
Top 10 Machine Learning Career Myths Debunked: Key Facts for Aspiring Professionals
Machine learning (ML) has become one of the hottest fields in technology—touching everything from recommendation engines and self-driving cars to language translation and healthcare diagnostics. The immense potential of ML, combined with attractive compensation packages and high-profile success stories, has spurred countless professionals and students to explore this career path. Yet, despite the boom in demand and innovation, machine learning is not exempt from myths and misconceptions. At MachineLearningJobs.co.uk, we’ve had front-row seats to the real-life career journeys and hiring needs in this field. We see, time and again, that outdated assumptions—like needing a PhD from a top university or that ML is purely about deep neural networks—can mislead new entrants and even deter seasoned professionals from making a successful transition. If you’re curious about a career in machine learning or looking to take your existing ML expertise to the next level, this article is for you. Below, we debunk 10 of the most persistent myths about machine learning careers and offer a clear-eyed view of the essential skills, opportunities, and realistic paths forward. By the end, you’ll be better equipped to make informed decisions about your future in this dynamic and rewarding domain.
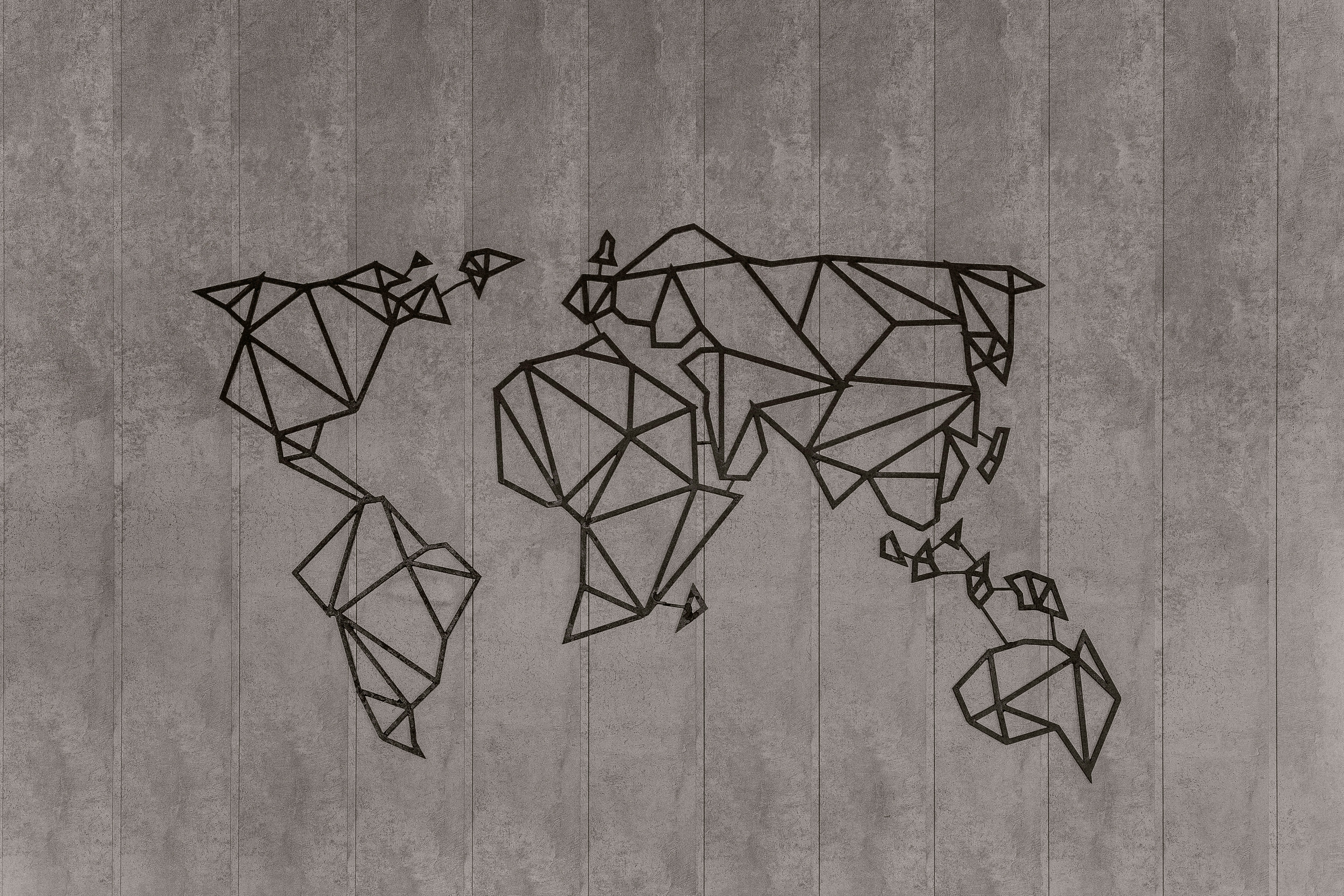
Global vs. Local: Comparing the UK Machine Learning Job Market to International Landscapes
How to evaluate opportunities, salaries, and work culture in machine learning across the UK, the US, Europe, and Asia Machine learning (ML) has rapidly transcended the research labs of academia to become a foundational pillar of modern technology. From recommendation engines and autonomous vehicles to fraud detection and personalised healthcare, machine learning techniques are increasingly ubiquitous, transforming how organisations operate. This surge in applications has fuelled an extraordinary global demand for ML professionals—data scientists, ML engineers, research scientists, and more. In this article, we’ll examine how the UK machine learning job market compares to prominent international hubs, including the United States, Europe, and Asia. We’ll explore hiring trends, salary ranges, workplace cultures, and the nuances of remote and overseas roles. Whether you’re a fresh graduate aiming to break into the field, a software engineer with an ML specialisation, or a seasoned professional seeking your next challenge, understanding the global ML landscape is essential for making an informed career move. By the end of this overview, you’ll be equipped with insights into which regions offer the best blend of salaries, work-life balance, and cutting-edge projects—plus practical tips on how to succeed in a domain that’s constantly evolving. Let’s dive in.