Related Jobs
View all jobsData Scientist
Data Scientist
Data Scientist
Data Scientist / AI Engineer (TensorFlow, PyTorch)
Data Scientist 80k
Get the latest insights and jobs direct. Sign up for our newsletter.
Industry Insights
Discover insightful articles, industry insights, expert tips, and curated resources.

Navigating Machine Learning Career Fairs Like a Pro: Preparing Your Pitch, Questions to Ask, and Follow-Up Strategies to Stand Out
Machine learning (ML) has swiftly become one of the most in-demand skill areas across industries, with companies leveraging predictive models and data-driven insights to solve challenges in healthcare, finance, retail, manufacturing, and beyond. Whether you’re an early-career data scientist aiming to break into ML, a seasoned engineer branching into deep learning, or a product manager exploring AI-driven solutions, machine learning career fairs offer a powerful route to connect with prospective employers face-to-face. Attending these events can help you: Network with hiring managers and technical leads who make direct recruitment decisions. Gain insider insights on the latest ML trends and tools. Learn about emerging job roles and new industry verticals adopting machine learning. Showcase your interpersonal and communication skills, both of which are increasingly important in collaborative AI/ML environments. However, with many applicants vying for attention in a bustling hall, standing out isn’t always easy. In this detailed guide, we’ll walk you through how to prepare meticulously, pitch yourself confidently, ask relevant questions, and follow up effectively to land the machine learning opportunity that aligns with your ambitions.
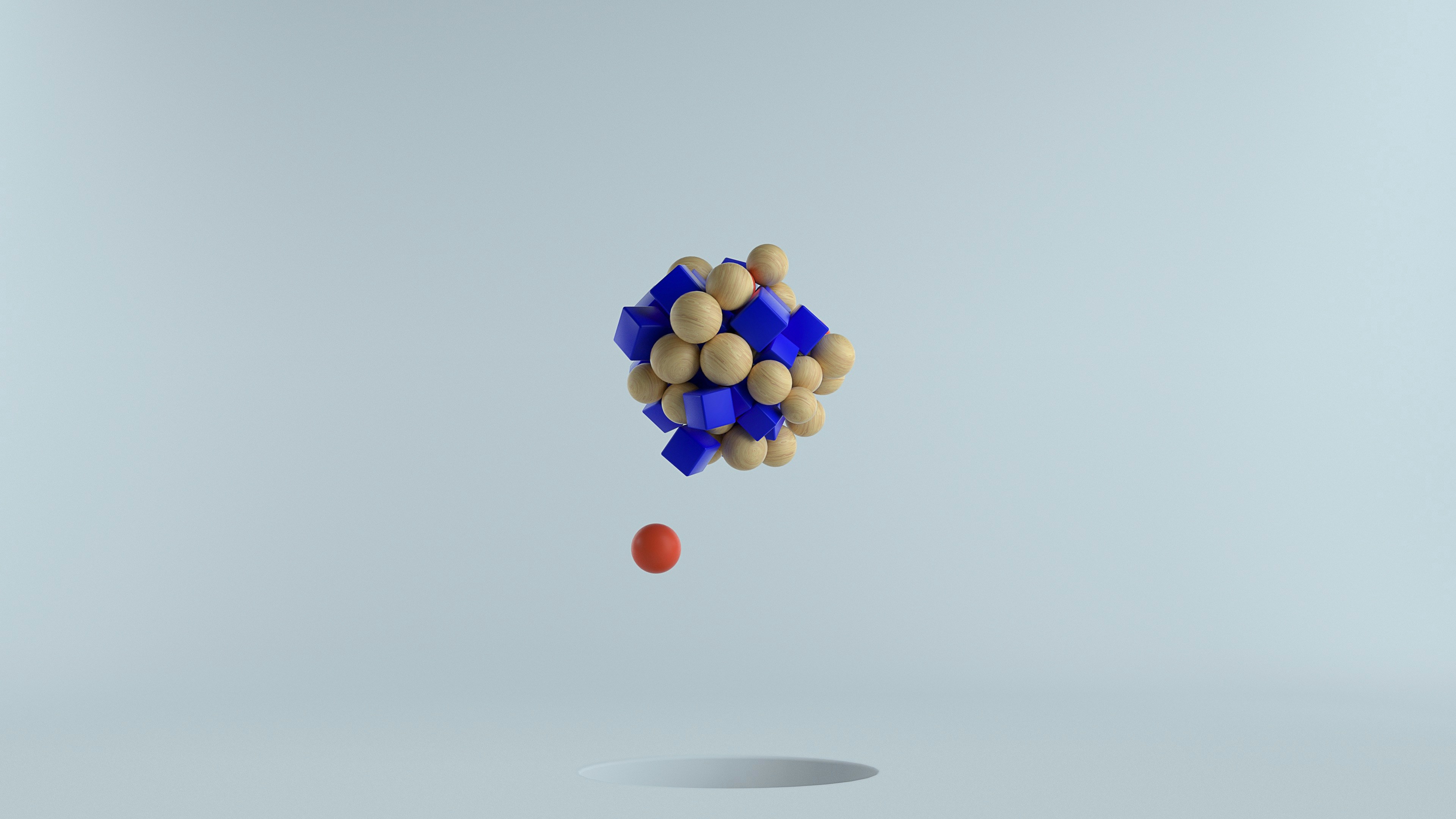
Common Pitfalls Machine Learning Job Seekers Face and How to Avoid Them
Machine learning has emerged as one of the most sought-after fields in technology, with companies across industries—from retail and healthcare to finance and manufacturing—embracing data-driven solutions at an unprecedented pace. In the UK, the demand for skilled ML professionals continues to soar, and opportunities in this domain are abundant. Yet, amid this growing market, competition for machine learning jobs can be fierce. Prospective employers set a high bar: they seek candidates with not just theoretical understanding, but also strong practical skills, business sense, and an aptitude for effective communication. Whether you’re a recent graduate, a data scientist transitioning into machine learning, or a seasoned developer pivoting your career, it’s essential to avoid common mistakes that may hinder your prospects. This blog post explores the pitfalls frequently encountered by machine learning job seekers, and offers actionable guidance on how to steer clear of them. If you’re looking for roles in this thriving sector, don’t forget to check out Machine Learning Jobs for the latest vacancies across the UK. In this article, we’ll break down these pitfalls to help you refine your approach in applications, interviews, and career development. By taking on board these insights, you can significantly enhance your employability, stand out from the competition, and secure a rewarding position in the world of machine learning.
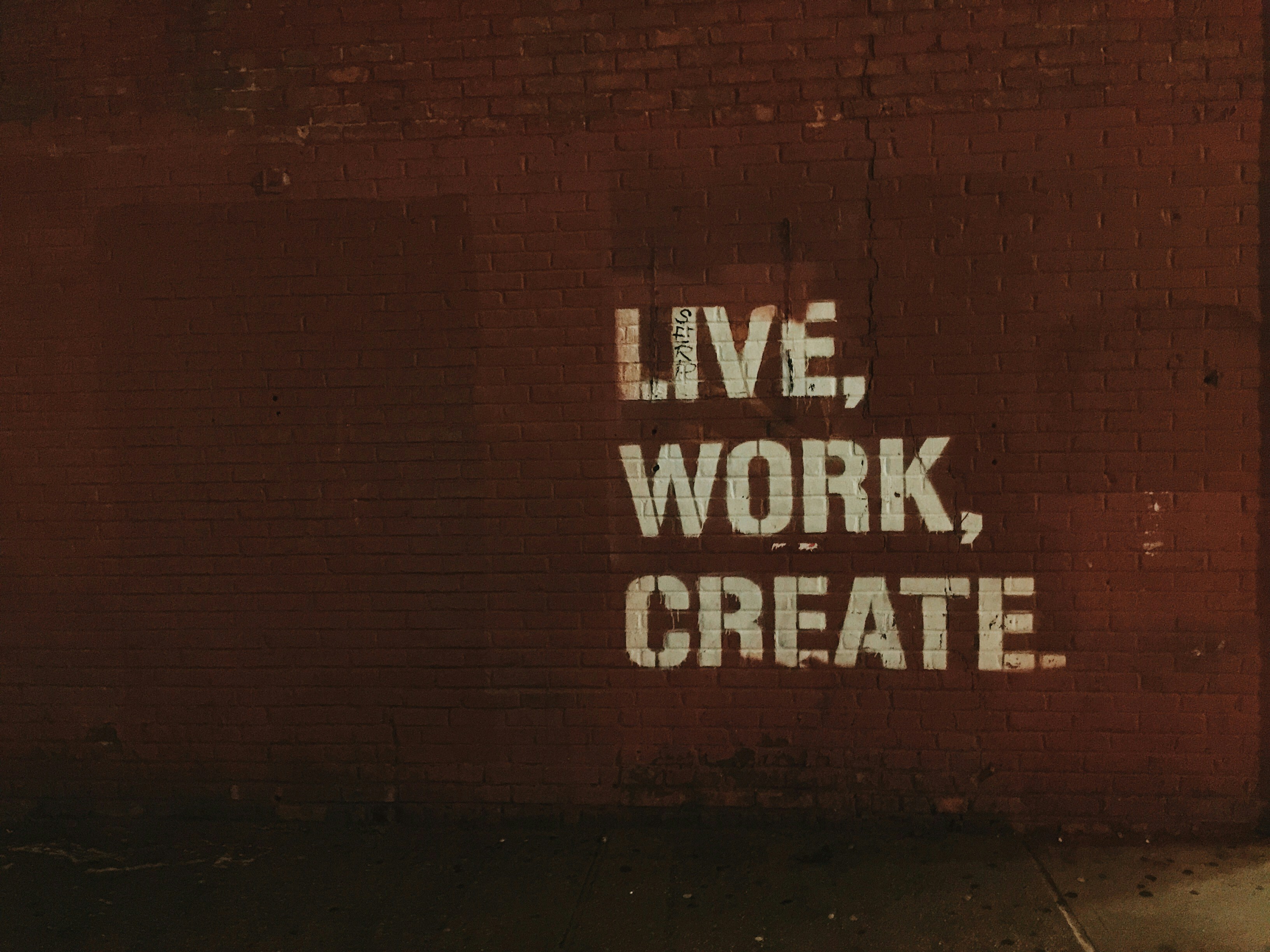
Career Paths in Machine Learning: From Entry-Level Roles to Leadership and Beyond
Machine learning has rapidly transformed from an academic pursuit to a cornerstone of modern technology, fueling innovations in healthcare, finance, retail, cybersecurity, and virtually every industry imaginable. From predictive analytics and computer vision to deep learning models that power personalisation algorithms, machine learning (ML) is reshaping business strategies and creating new economic opportunities. As demand for ML expertise continues to outstrip supply, the UK has become a vibrant hub for machine learning research, entrepreneurship, and corporate adoption. Whether you’re just starting out or have experience in data science, software development, or adjacent fields, there has never been a better time to pursue a career in machine learning. In this article, we will explore: The growing importance of machine learning in the UK Entry-level roles that can kick-start your ML career The skills and qualifications you’ll need to succeed Mid-level and advanced positions, including leadership tracks Tips for job seekers on www.machinelearningjobs.co.uk By the end, you’ll have a clear view of how to build, grow, and lead in one of the most exciting fields in modern technology.