Related Jobs
View all jobsPrincipal Data Scientist - NLP
Head of Analytics and AI (Basé à London)
Head of Analytics and AI
Director, Onyx Product Engagement
Applied AI Engineer
Get the latest insights and jobs direct. Sign up for our newsletter.
Industry Insights
Discover insightful articles, industry insights, expert tips, and curated resources.
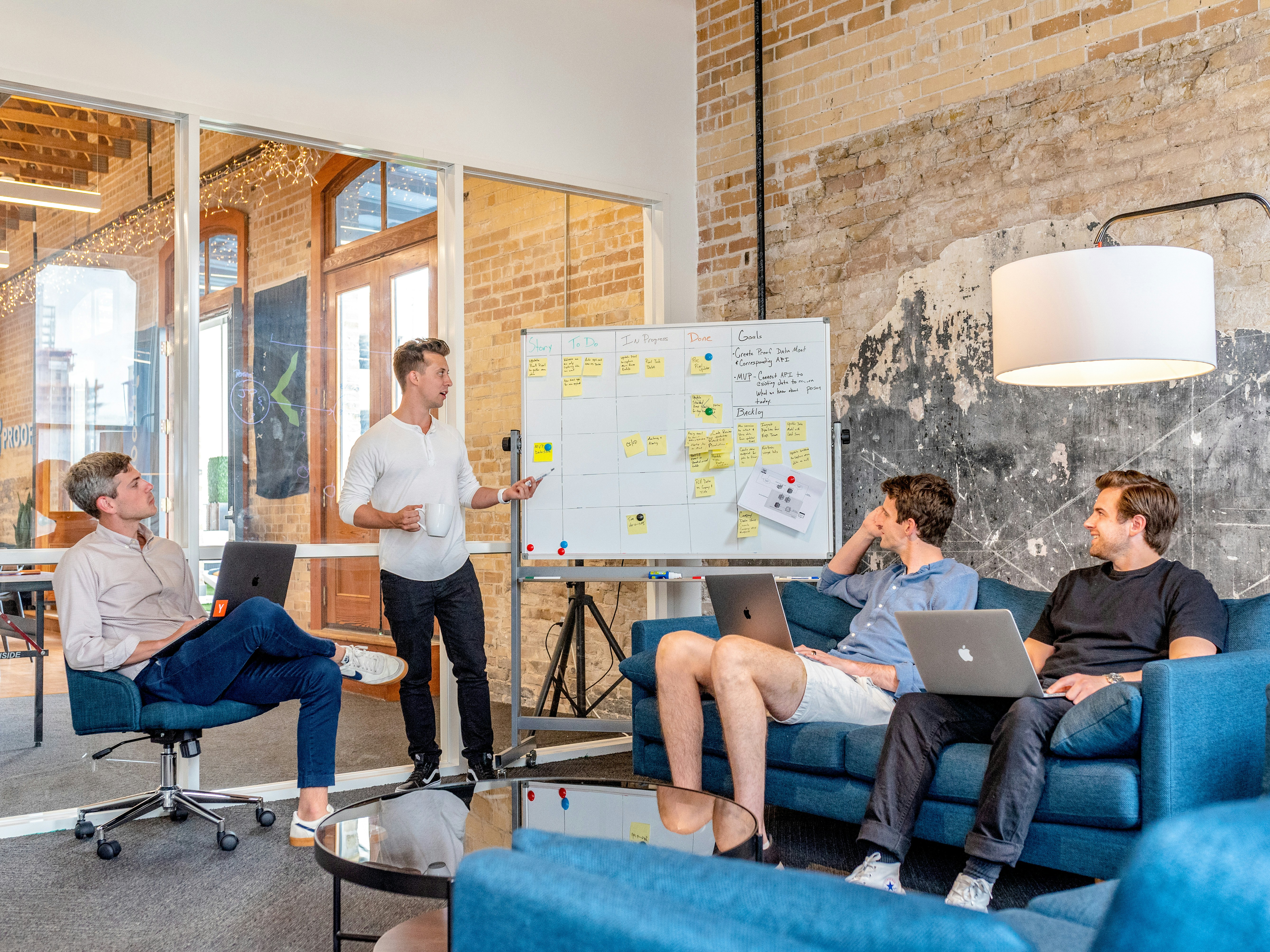
Machine‑Learning Jobs for Non‑Technical Professionals: Where Do You Fit In?
The Model Needs More Than Math When ChatGPT went viral and London start‑ups raised seed rounds around “foundation models,” many professionals asked, “Do I need to learn PyTorch to work in machine learning?” The answer is no. According to the Turing Institute’s UK ML Industry Survey 2024, 39 % of advertised ML roles focus on strategy, compliance, product or operations rather than writing code. As models move from proof‑of‑concept to production, demand surges for specialists who translate algorithms into business value, manage risk and drive adoption. This guide reveals the fastest‑growing non‑coding ML roles, the transferable skills you may already have, real transition stories and a 90‑day action plan—no gradient descent necessary.
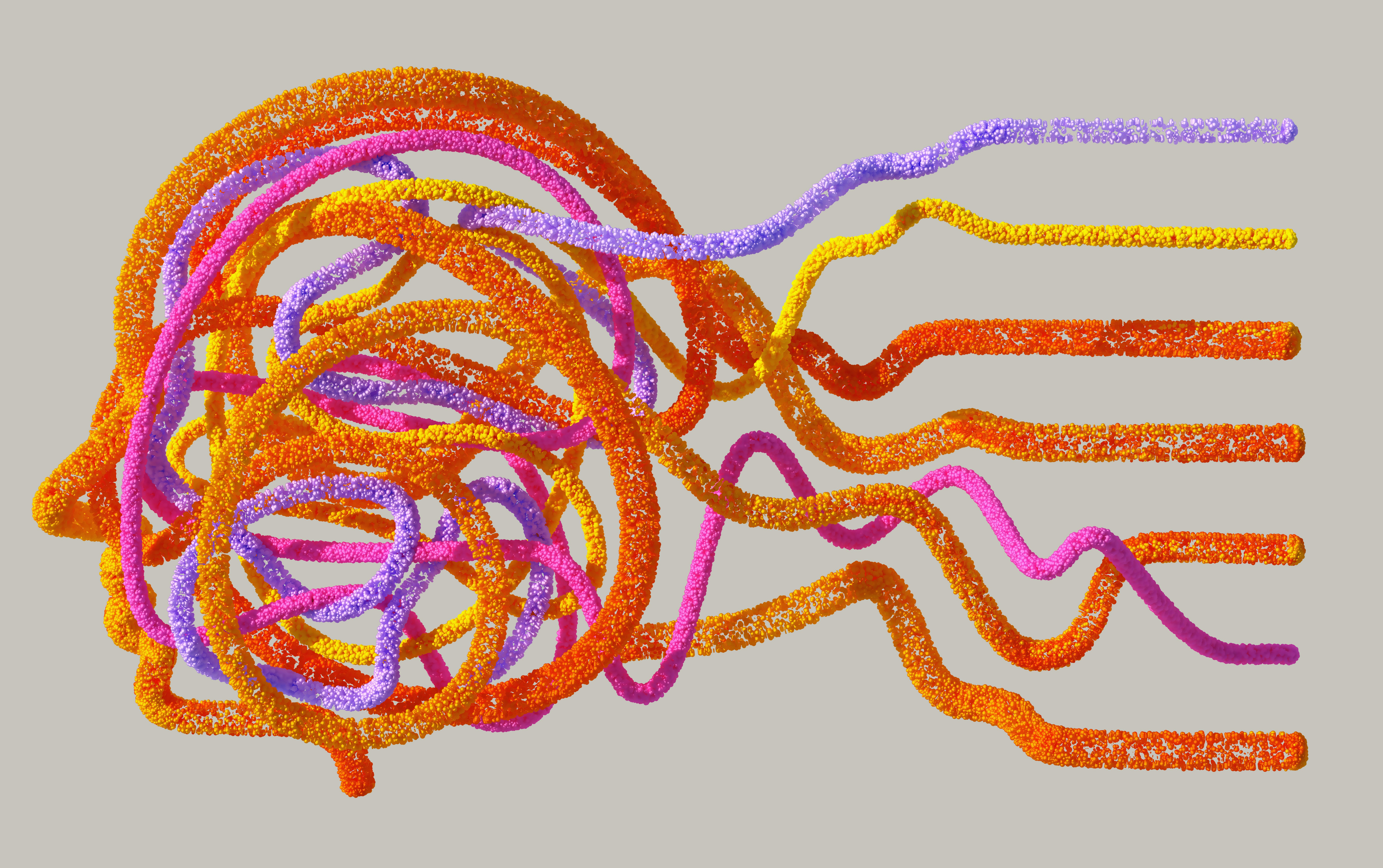
Quantexa Machine‑Learning Jobs in 2025: Your Complete UK Guide to Joining the Decision‑Intelligence Revolution
Money‑laundering rings, sanctioned entities, synthetic identities—complex risks hide in plain sight inside data. Quantexa, a London‑born scale‑up now valued at US $2.2 bn (Series F, August 2024), solves that problem with contextual decision‑intelligence (DI): graph analytics, entity resolution and machine learning stitched into a single platform. Banks, insurers, telecoms and governments from HSBC to HMRC use Quantexa to spot fraud, combat financial crime and optimise customer engagement. With the launch of Quantexa AI Studio in February 2025—bringing generative AI co‑pilots and large‑scale Graph Neural Networks (GNNs) to the platform—the company is hiring at record pace. The Quantexa careers portal lists 450+ open roles worldwide, over 220 in the UK across data science, software engineering, ML Ops and client delivery. Whether you are a graduate data scientist fluent in Python, a Scala veteran who loves Spark or a solutions architect who can turn messy data into knowledge graphs, this guide explains how to land a Quantexa machine‑learning job in 2025.

Machine Learning vs. Deep Learning vs. MLOps Jobs: Which Path Should You Choose?
Machine Learning (ML) continues to transform how businesses operate, from personalised product recommendations to automated fraud detection. As ML adoption accelerates in nearly every industry—finance, healthcare, retail, automotive, and beyond—the demand for professionals with specialised ML skills is surging. Yet as you browse Machine Learning jobs on www.machinelearningjobs.co.uk, you may encounter multiple sub-disciplines, such as Deep Learning and MLOps. Each of these fields offers unique challenges, requires a distinct skill set, and can lead to a rewarding career path. So how do Machine Learning, Deep Learning, and MLOps differ? And which area best aligns with your talents and aspirations? This comprehensive guide will define each field, highlight overlaps and differences, discuss salary ranges and typical responsibilities, and explore real-world examples. By the end, you’ll have a clearer vision of which career track suits you—whether you prefer building foundational ML models, pushing the boundaries of neural network performance, or orchestrating robust ML pipelines at scale.