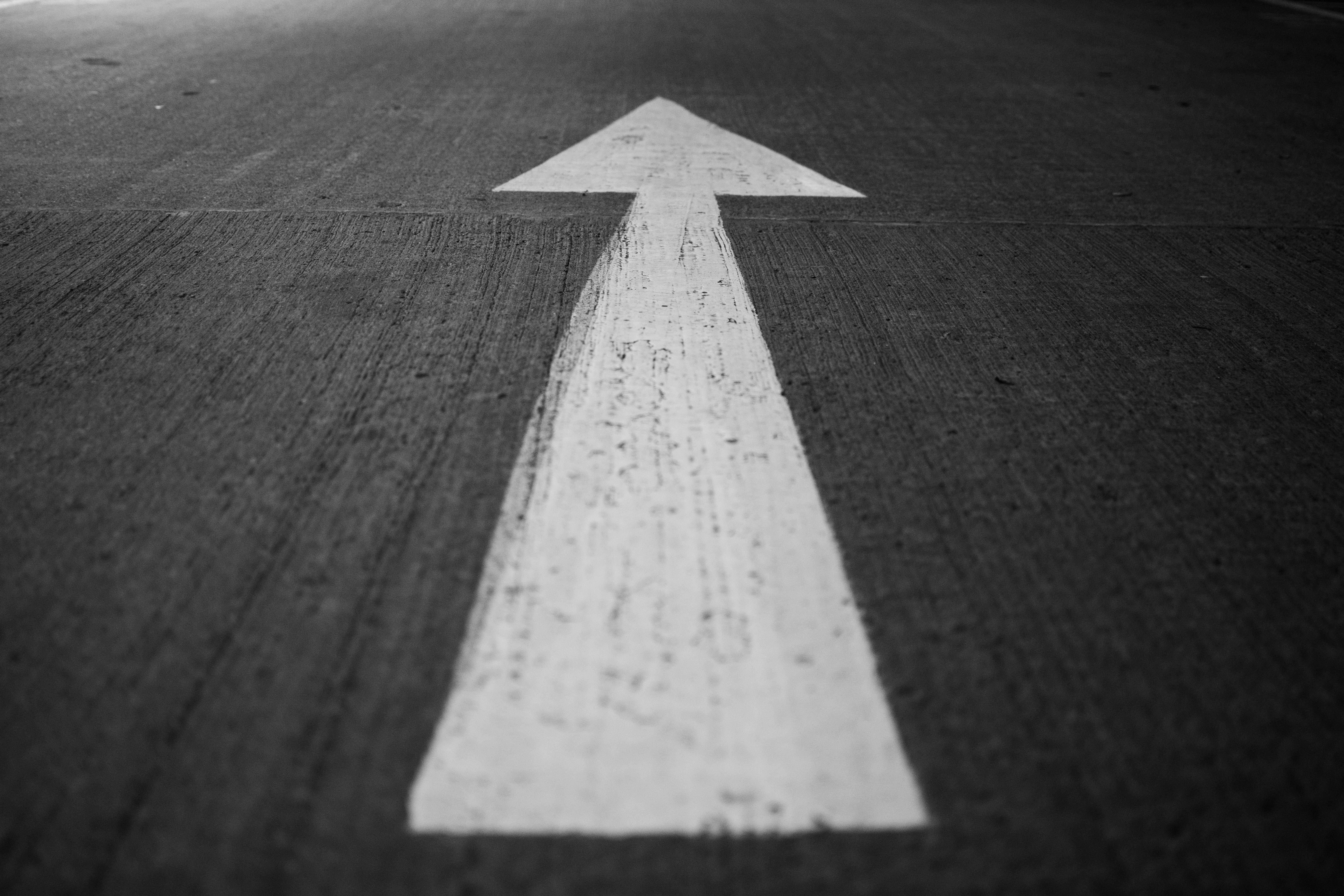
Machine Learning Sector Predictions for the Next 5 Years: Technological Progress, Emerging Applications, and the Evolving Job Market
Machine Learning (ML) has become one of the defining technologies of our age, enabling breakthroughs across diverse sectors—from healthcare and finance to retail and entertainment. As data volumes continue to grow exponentially, businesses are racing to leverage algorithms that can derive valuable insights, automate tedious tasks, and unlock new revenue streams. With this momentum, the UK stands at the forefront of Machine Learning innovation—home to world-class research institutions, well-funded tech start-ups, and established enterprises that recognise ML’s transformative potential.
In this article, we explore key Machine Learning (ML) sector predictions for the next five years, looking at the technological advances reshaping the field, the most promising industry applications, and the evolving job market in the UK. Whether you’re an aspiring data scientist, a seasoned ML engineer, or simply curious about the future of AI and ML, understanding these trends can help you position yourself for success in a rapidly changing landscape.
1. The Expanding Influence of Machine Learning in the UK
From consumer-facing apps and recommendation systems to large-scale data analytics in financial services, the influence of Machine Learning is widespread and growing. The UK is often considered a major European technology hub, with governmental bodies, private corporations, and academic institutions investing significantly in both fundamental research and commercial deployments.
1.1 Government Initiatives and R&D Investments
The UK government has launched multiple programmes supporting AI and ML research, including funding for cutting-edge research centres, AI scholarships, and the development of national data strategies. These initiatives aim to:
Foster Collaboration: Encouraging partnerships between universities, start-ups, and established tech players.
Boost Skills and Training: Addressing the shortage of ML specialists through grants, fellowships, and scholarships, ensuring a steady flow of new talent.
Enhance International Competitiveness: Positioning the UK as a global leader in high-growth areas like healthcare AI, FinTech, and autonomous systems.
1.2 Private Sector and Start-up Ecosystem
Beyond government support, the UK’s flourishing start-up ecosystem is accelerating ML adoption. London’s “Silicon Roundabout,” Cambridge’s “Silicon Fen,” and Manchester’s tech clusters have witnessed a surge of ventures applying Machine Learning to areas like natural language processing (NLP), computer vision, predictive analytics, and more. The next five years will see:
Increased VC Funding: More venture capitalists and angel investors are backing ML-focused start-ups, driving job creation and innovation.
Corporate Adoption: Enterprises in finance, retail, telecoms, and other verticals will increasingly integrate ML solutions to streamline operations and differentiate themselves in competitive markets.
2. Key Technological Trends for the Next Five Years
Machine Learning is a dynamic field, with new frameworks, algorithms, and hardware optimisations emerging rapidly. Below, we take a closer look at the trends set to define ML’s trajectory in the near future.
2.1 MLOps and Scalable AI Pipelines
As more organisations transition from ML proof-of-concepts to full-scale deployments, MLOps (Machine Learning Operations) is becoming critical. MLOps integrates DevOps best practices into ML workflows, ensuring models are developed, tested, deployed, and monitored in a repeatable, reliable manner. Over the next five years, expect:
End-to-End Platforms: Toolchains that unify data ingestion, model training, version control, deployment, and monitoring—offered by major cloud providers (e.g., AWS Sagemaker, Azure ML, GCP Vertex AI).
Model Monitoring & Feedback Loops: Real-time detection of model drift, performance anomalies, and data quality issues, coupled with automated re-training pipelines.
Cross-Functional Collaboration: Closer alignment between data scientists, software engineers, and IT operations as businesses scale ML across multiple products and departments.
2.2 Low-Code / No-Code ML Solutions
While demand for skilled ML engineers remains high, low-code and no-code ML platforms are lowering barriers to entry for non-experts. By offering drag-and-drop interfaces, auto-ML functionalities, and pre-built connectors, these solutions enable business analysts or domain specialists to:
Prototype Quickly: Train and deploy models without writing extensive code.
Focus on Use Cases: Spend more time defining business metrics and less time wrestling with technical complexity.
Democratise AI: Expand ML capabilities beyond data science teams, fostering a culture of experimentation.
Over the next five years, these user-friendly tools will proliferate, creating new pathways for citizen data scientists while complementing, rather than replacing, advanced ML skill sets.
2.3 TinyML and Edge Computing
As the Internet of Things (IoT) expands, TinyML—running ML algorithms on low-power, resource-constrained devices—will gain momentum. Advances in hardware accelerators and model compression techniques are enabling real-time inference directly on embedded devices. This shift brings:
Low Latency: Vital for applications like autonomous drones, industrial robotics, and AR/VR, where split-second decisions are required.
Reduced Bandwidth Usage: Processing data on the device means less reliance on cloud connectivity.
Privacy Benefits: Sensitive data stays local, mitigating security and compliance risks.
The growing intersection of edge computing and ML opens roles for embedded systems engineers, sensor data analysts, and MLOps professionals versed in distributed architectures.
2.4 Transfer Learning and Pre-Trained Models
One of ML’s biggest breakthroughs in recent years has been transfer learning, where a model pre-trained on large datasets is fine-tuned for a specific task with relatively little data. Pre-trained models in NLP (e.g., GPT-like architectures) and computer vision (e.g., ResNet, EfficientNet) have become standard practice, accelerating model development. Over the next five years:
Domain-Specific Models: Expect more pre-trained models tailored for healthcare, finance, cybersecurity, and beyond.
Model Hubs and Repositories: Platforms like Hugging Face or TensorFlow Hub will expand, allowing developers to mix and match pre-trained architectures easily.
Performance vs. Explainability: As models grow more complex, balancing raw accuracy with interpretability and compliance becomes an ongoing challenge.
2.5 Responsible and Explainable AI
With ML systems increasingly affecting crucial decisions—loan approvals, medical diagnoses, recruitment—ethical and explainable AI will be top of mind. The next five years will see stronger emphasis on:
Bias Mitigation: Techniques to detect and reduce unfair bias in training data or model predictions.
Model Explainability: Greater demand for interpretable ML approaches, especially in regulated sectors like finance and healthcare.
AI Governance & Compliance: More organisations adopting frameworks for AI risk management, audit trails, and regulatory alignment.
Professionals with a mix of ML expertise and ethical or legal insights will find themselves in high demand, shaping how ML is deployed responsibly.
3. Industry Applications and Growth Areas
Machine Learning now underpins many day-to-day technologies—recommendation engines, voice assistants, fraud detection systems—and is expanding rapidly into new domains. Below are some of the most promising industry applications set to flourish in the next five years.
3.1 Healthcare and Life Sciences
ML-driven healthcare solutions are already improving diagnostics, treatment recommendations, and patient care management. Expect deeper transformations as data from patient records, wearables, and genomics multiply:
Personalised Medicine: Advanced predictive models that tailor drug regimens or treatment paths based on individual risk profiles.
Medical Imaging: Computer vision tools to detect anomalies in X-rays, MRIs, or CT scans faster and, in some cases, more accurately than human radiologists.
Drug Discovery: ML algorithms accelerating research pipelines by predicting molecular interactions and potential side effects.
Career-wise, roles bridging data science with biomedical or genomic expertise will multiply, alongside regulatory-compliance analysts ensuring patient data privacy (e.g., GDPR) is maintained.
3.2 Financial Services and FinTech
The financial sector has long been an avid adopter of data analytics, but ML promises a new level of precision and automation:
Algorithmic Trading: Models capable of analysing massive datasets in real time, identifying trading opportunities or detecting market manipulation patterns.
Fraud Detection: Real-time monitoring of transactions for suspicious patterns—credit card anomalies, identity theft—using advanced anomaly detection techniques.
Credit Scoring: More granular models that account for alternative data (e.g., social media behaviour) to assess creditworthiness, while also maintaining fairness and transparency.
With London being a global financial hub, ML-related roles in FinTech, risk management, and compliance are poised for continued growth.
3.3 Retail and Marketing
The retail sector uses ML to personalise customer experiences, optimise supply chains, and manage dynamic pricing. As consumer expectations rise:
Recommendation Engines: ML algorithms powering product suggestions, upselling, and cross-selling strategies.
Inventory Forecasting: Predictive analytics to manage supply chains, preventing stock shortages or overstock scenarios.
Customer Segmentation: Clustering and classification techniques that group customers by behaviour or preferences, enabling targeted campaigns and loyalty programmes.
Demand for data-driven marketers, ML-savvy merchandisers, and e-commerce optimisation specialists will expand as online and offline retail converge.
3.4 Manufacturing and Industry 4.0
Manufacturers are harnessing ML to increase efficiency, reduce downtime, and enhance product quality. Over the next five years:
Predictive Maintenance: IoT sensors feeding real-time data into ML models that predict machine failures before they happen.
Quality Control: Automated inspection systems using computer vision to identify defects or anomalies in production lines.
Robotics & Cobots: Machine Learning-infused robots that can adapt to complex tasks, sometimes working alongside human operators.
These trends call for ML specialists with knowledge of industrial automation, embedded computing, and cyber-physical systems.
3.5 Autonomous Systems and Mobility
Self-driving cars, delivery drones, and autonomous robots are already making headlines. While fully autonomous vehicles face technical and regulatory challenges, the next five years will see:
Incremental Autonomy: Assisted driving and driver-monitoring systems become standard in new vehicles, reducing road accidents.
Swarm Robotics: Coordinated fleets of drones and robots for warehouse logistics, surveillance, or disaster relief.
Smart Infrastructure: Traffic light systems and public transport networks leveraging ML to optimise flows and reduce congestion.
Roles in computer vision, sensor fusion, reinforcement learning, and safety engineering will be central to the growth of autonomous systems.
4. The Evolving Machine Learning Job Market in the UK
Given the explosive demand for ML-driven solutions, the UK job market continues to evolve. Employers are looking for talent that blends technical mastery with industry-specific knowledge, communication skills, and adaptability.
4.1 In-Demand Roles
ML Engineer
Skilled in building scalable machine learning pipelines, implementing models into production, and managing associated cloud infrastructure. Proficiency in Python, TensorFlow/PyTorch, Docker, and Kubernetes is often essential.Data Scientist
Responsible for data analysis, feature engineering, and prototype model development. Strong statistics background, domain expertise, and communication skills for presenting findings to stakeholders.NLP Specialist
Focused on developing language models, chatbots, and text analytics solutions. Advanced knowledge of linguistics and popular NLP libraries (e.g., spaCy, Hugging Face, NLTK) is crucial.Computer Vision Engineer
Experts in image processing and object detection, crucial for autonomous vehicles, surveillance systems, and medical imaging solutions.Machine Learning Researcher
Those pushing the boundaries of ML theory—exploring new architectures, optimisation techniques, or novel applications. Often found at cutting-edge research labs or tech giants.AI Product Manager
The bridge between technical teams and business objectives. Oversees product roadmaps, ensuring ML solutions align with customer needs, compliance requirements, and commercial goals.
4.2 Remote and Hybrid Work Opportunities
Many ML projects—from data wrangling to model training—can be conducted remotely, provided secure data access is in place. This flexibility broadens the talent pool for UK-based companies, allowing them to attract experts from different geographies or retain talent that prefers hybrid work arrangements. Consequently:
Distributed Teams: Collaboration platforms, version control, and MLOps tools are standard, enabling seamless teamwork across locations.
Flexible Schedules: As ML tasks can sometimes be asynchronous (training models overnight, for instance), employees enjoy greater autonomy in time management.
4.3 Salary Trends and Competition
Salaries for ML roles in the UK often reflect the scarcity of top-tier talent, especially in London and other tech hubs. Data scientists and ML engineers can command competitive packages, and as the demand continues to outstrip supply, compensation levels are likely to remain high. Companies are also offering equity or bonus schemes to attract and retain talent. Beyond pure salary, the opportunity to work on high-impact, innovative projects is a major draw.
5. How Technology Progress Will Shape Roles
The synergy between Machine Learning and other emerging technologies—IoT, blockchain, quantum computing—will amplify ML’s transformative potential. Below are some developments that will shape roles and skills in the near future.
5.1 Quantum Computing and ML
While still nascent, quantum computing could revolutionise ML by accelerating large-scale training tasks and complex optimisation problems. In the next few years:
Hybrid Quantum-Classical Approaches: Early demonstration of quantum circuits boosting certain ML tasks.
Quantum Machine Learning (QML): Exploratory roles for researchers who straddle quantum physics and deep learning.
Specialised Tooling: Growth in software ecosystems (e.g., Qiskit, Cirq) bridging quantum hardware and classical ML frameworks.
5.2 Blockchain and Federated Learning
Federated learning allows model training on decentralised data sources without requiring raw data to leave local devices. Combined with blockchain, this approach can provide secure, tamper-proof records of training events and model updates. Potential use cases include:
Healthcare Data Sharing: Safely aggregating insights from multiple hospitals while preserving patient privacy.
Finance Consortia: Banks collaborating on shared ML models without exposing sensitive transactional data.
IoT Networks: Edge devices training local models, then contributing updates to a global model on the blockchain.
Professionals adept at cryptography, distributed systems, and ML will find increasing opportunities as these solutions mature.
5.3 Metaverse and Extended Reality
The metaverse concept, driven by advances in VR/AR and real-time 3D graphics, could create new frontiers for ML:
Virtual Assistants and Avatars: NPCs (Non-Player Characters) or digital humans that interact naturally with users, powered by large language models and advanced speech synthesis.
Real-Time Analytics: ML-based analysis of user behaviour and environment data within VR/AR worlds, enabling dynamic content adaptation or safety measures.
Spatial Computing: Integration of computer vision, sensor fusion, and ML for realistic object detection and environment mapping.
While still largely experimental, growth in this space will see increased demand for developers with ML, 3D graphics, and real-time systems expertise.
6. Preparing for a Machine Learning Career: Skills and Strategies
To capitalise on the burgeoning ML job market, prospective candidates should develop well-rounded skill sets. Here are some strategies:
6.1 Technical Foundations
Programming Languages: Python remains the go-to for most ML tasks, but R, Java, and C++ can also be beneficial.
ML Frameworks: Proficiency with TensorFlow, PyTorch, or scikit-learn is essential.
Mathematics and Statistics: Solid grounding in linear algebra, calculus, probability, and statistical modelling.
Data Engineering Skills: Familiarity with SQL, NoSQL databases, and distributed computing (e.g., Spark) can be a differentiator.
6.2 Soft Skills and Business Acumen
Communication: Explaining complex ML concepts to non-technical stakeholders is critical.
Critical Thinking: Identifying relevant metrics, challenging assumptions, and validating models with real-world constraints.
Domain Knowledge: Depth in a specific sector (healthcare, finance, retail, etc.) can differentiate you from generalist ML practitioners.
6.3 Continuous Learning and Networking
Online Courses & Bootcamps: Platforms like Coursera, edX, and specialised AI schools offer up-to-date courses on ML topics.
Conferences & Meetups: Events like the AI Summit in London or local ML/AI gatherings provide networking and learning opportunities.
Hackathons & Competitions: Websites such as Kaggle, DrivenData, or corporate hackathons help sharpen your skills and build a project portfolio.
6.4 Ethical and Regulatory Awareness
With regulators paying closer attention to AI and ML, especially in high-stakes domains, professionals who understand GDPR, bias detection, data privacy, and explainable AI will be invaluable.
7. Challenges and Considerations for the Next Five Years
While Machine Learning’s outlook is undeniably bright, it faces challenges:
7.1 Skills Shortage
Demand for ML talent outstrips supply. Employers may struggle to recruit specialists with both deep technical expertise and the soft skills needed to collaborate across teams.
7.2 Data Quality and Availability
ML models are only as good as the data they’re trained on. Companies must invest in data engineering, governance, and cleaning to ensure robust insights.
7.3 Regulatory and Ethical Complexities
As ML systems take on more responsibilities, legal liabilities and ethical implications rise. Companies must navigate a maze of regulations, from consumer protection laws to sector-specific compliance.
7.4 Rapid Technological Shifts
New frameworks, libraries, and paradigms emerge frequently, requiring ML professionals to continuously learn and adapt. Businesses also risk technology lock-in if they invest in proprietary tools that quickly become obsolete.
8. Conclusion: Embracing ML’s Transformative Potential
Machine Learning has already reshaped entire industries, and its influence will only grow as computational power increases, data sets expand, and new applications emerge. Over the next five years, we can expect:
Proliferation of MLOps: Seamless, scalable AI pipelines becoming standard in organisations large and small.
Expansion of Low-Code AI Tools: Democratising ML for non-technical stakeholders, driving broader adoption.
Integration with Edge and IoT: Real-time, on-device inference powering a new generation of autonomous systems.
Continued Emphasis on Explainability and Ethics: Balancing performance with societal responsibilities.
Cross-Pollination with Emerging Tech: ML blending with quantum computing, blockchain, and the metaverse to unlock novel solutions.
For job seekers, the next half-decade offers abundant opportunities to innovate, solve complex problems, and drive business value. By cultivating strong technical foundations, developing communication and domain skills, and staying attuned to ethical considerations, you can position yourself at the forefront of Machine Learning’s most exciting era yet—right here in the UK.
Explore Machine Learning Career Opportunities
Ready to take the next step? Visit www.machinelearningjobs.co.uk for the latest Machine Learning job postings across the UK. From entry-level data analyst roles to senior ML engineer positions, our platform connects you with cutting-edge companies shaping the future of AI and ML.
As the industry’s potential continues to unfold, there’s never been a more auspicious time to pursue a career in Machine Learning. Seize the moment, build your skills, and be part of the innovation driving tomorrow’s economy—today.