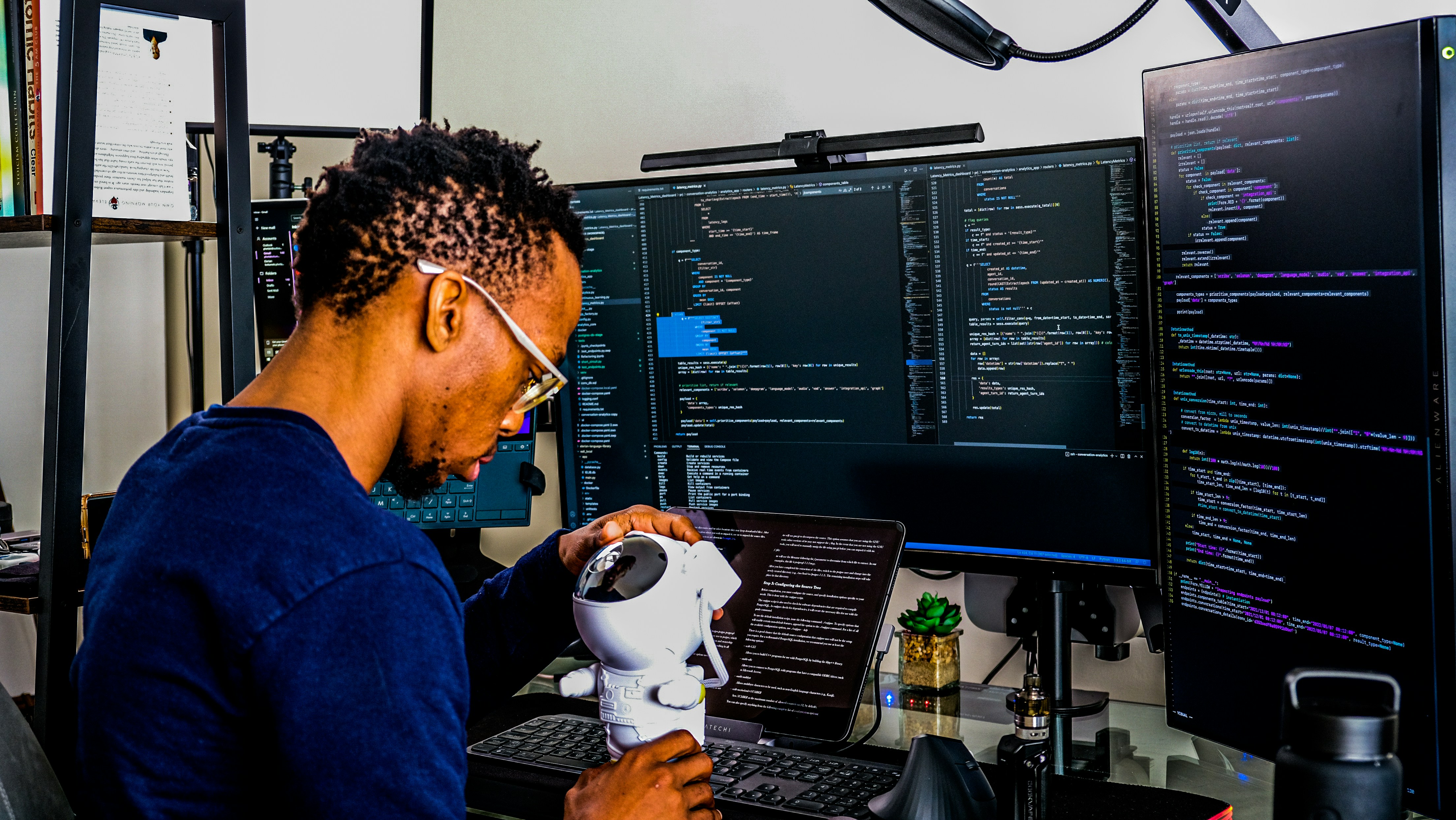
How to Excel as an Applied Machine Learning Researcher: Essential Skills for Career Growth
Machine learning continues to revolutionise industries, driving innovations in healthcare, finance, technology, and beyond. At the heart of these advancements are Applied Machine Learning Researchers, professionals who use machine learning techniques to solve real-world problems. If you’re aiming to excel in this highly specialised role, understanding the key skills and strategies for success is crucial. This article explores what it takes to stand out as an Applied Machine Learning Researcher and advance your career in this dynamic field.
What Does an Applied Machine Learning Researcher Do?
An Applied Machine Learning Researcher focuses on creating, testing, and implementing machine learning models to address practical challenges. They work at the intersection of cutting-edge research and application, bridging the gap between theoretical advancements and industry needs. Key responsibilities include:
Identifying and defining problems that can be solved with machine learning.
Designing and developing machine learning models.
Conducting experiments to evaluate model performance.
Collaborating with cross-functional teams to integrate models into production.
Staying updated with the latest research to inform practical applications.
This role requires a mix of research expertise, programming skills, and domain knowledge.
Core Technical Skills
To succeed as an Applied Machine Learning Researcher, you need a strong technical foundation. Key technical skills include:
1. Machine Learning and Deep Learning
Proficiency in supervised, unsupervised, and reinforcement learning techniques.
Expertise in deep learning architectures like CNNs, RNNs, and transformers.
Familiarity with frameworks such as TensorFlow, PyTorch, and Scikit-learn.
2. Programming Proficiency
Strong coding skills in Python, R, or Julia for implementing models.
Proficiency in C++ or Java for high-performance applications.
3. Mathematics and Statistics
Solid understanding of linear algebra, calculus, probability, and statistics.
Ability to apply mathematical concepts to optimise machine learning models.
4. Data Engineering
Experience in data preprocessing, feature engineering, and data cleaning.
Familiarity with tools like Apache Spark, Hadoop, or AWS for handling large datasets.
Research and Analytical Skills
Applied Machine Learning Researchers must be adept at bridging the gap between theoretical research and practical implementation. Key skills include:
1. Problem-Solving and Creativity
Identifying innovative ways to apply machine learning to real-world problems.
Designing experiments to validate new approaches.
2. Critical Thinking
Evaluating the strengths and limitations of various models.
Analysing the trade-offs between model complexity and performance.
3. Staying Current with Research
Reading and understanding academic papers.
Implementing state-of-the-art techniques from recent publications.
Soft Skills
While technical expertise is crucial, soft skills are equally important for excelling in this role:
1. Collaboration and Teamwork
Working closely with data scientists, engineers, and product managers.
Sharing knowledge and mentoring junior team members.
2. Communication Skills
Explaining complex models and results to non-technical stakeholders.
Writing clear and comprehensive documentation.
3. Adaptability
Navigating the rapidly evolving field of machine learning.
Adjusting models and strategies in response to feedback or new findings.
Educational Background and Certifications
A strong academic background and certifications can significantly enhance your credibility as an Applied Machine Learning Researcher:
1. Degrees
A Bachelor’s degree in Computer Science, Mathematics, or a related field is typically required.
A Master’s or PhD in Machine Learning, AI, or Data Science can provide a competitive edge, especially for research-intensive roles.
2. Certifications
TensorFlow Developer Certificate: Validates your expertise in deep learning.
AWS Certified Machine Learning - Specialty: Highlights your ability to build and deploy machine learning models on AWS.
Coursera or edX Certifications: Courses in deep learning, NLP, or computer vision from leading universities.
Tools and Technologies
Proficiency in a variety of tools and technologies is essential for applied research in machine learning:
1. Machine Learning Frameworks
TensorFlow, PyTorch, Keras for building models.
OpenCV for computer vision applications.
2. Data Tools
Pandas and NumPy for data manipulation.
Apache Spark or Dask for big data processing.
3. Development Environments
Jupyter Notebook for prototyping and experimentation.
PyCharm or VS Code for software development.
4. Version Control
Git and GitHub for managing code and collaborating with teams.
How to Stand Out as an Applied Machine Learning Researcher
To differentiate yourself in the competitive machine learning job market, consider these strategies:
1. Build a Strong Research Portfolio
Showcase projects that highlight your ability to solve practical problems using machine learning.
Include case studies and code repositories on platforms like GitHub.
2. Contribute to Open Source
Collaborate on open-source machine learning projects to gain experience and visibility.
Publish your own tools or libraries to demonstrate expertise.
3. Stay Updated on Trends
Follow conferences like NeurIPS, CVPR, and ICML to stay informed about the latest research.
Read preprints on platforms like arXiv to explore emerging ideas.
4. Tailor Your CV
Use keywords such as “machine learning research,” “deep learning,” and “model optimisation.”
Highlight measurable achievements, e.g., “Improved model accuracy by 15% using advanced hyperparameter tuning.”
5. Polish Your Interview Skills
Prepare to discuss:
How you’ve applied machine learning to solve specific problems.
Your approach to staying updated with the latest research.
Challenges you’ve faced in implementing complex models.
Real-World Examples of Success
Understanding how others have excelled can provide valuable insights. Here are a few hypothetical scenarios:
Case Study 1: Developed a deep learning model for detecting diseases in medical images, improving diagnostic accuracy by 20%.
Case Study 2: Created a recommendation system for an e-commerce platform, increasing user engagement by 25%.
Case Study 3: Implemented a natural language processing pipeline for customer sentiment analysis, reducing churn rates by 15%.
Final Thoughts
The role of an Applied Machine Learning Researcher is both challenging and rewarding, offering the opportunity to bridge the gap between research and application. By developing the right mix of technical, research, and soft skills, you can position yourself as a standout candidate in this high-demand field.
Whether you’re starting your career or looking to advance, this guide provides a roadmap to success. For more career advice and job opportunities in machine learning, visit Machine Learning Jobs.