Related Jobs
View all jobsSenior Applied Scientist, Computer Vision, Camera and Sensors
Principal Software Engineer
Data Engineer - SC Clearance
Principal Security Data Analyst
[Apply Now] Principal Data Scientist...
Get the latest insights and jobs direct. Sign up for our newsletter.
Industry Insights
Discover insightful articles, industry insights, expert tips, and curated resources.
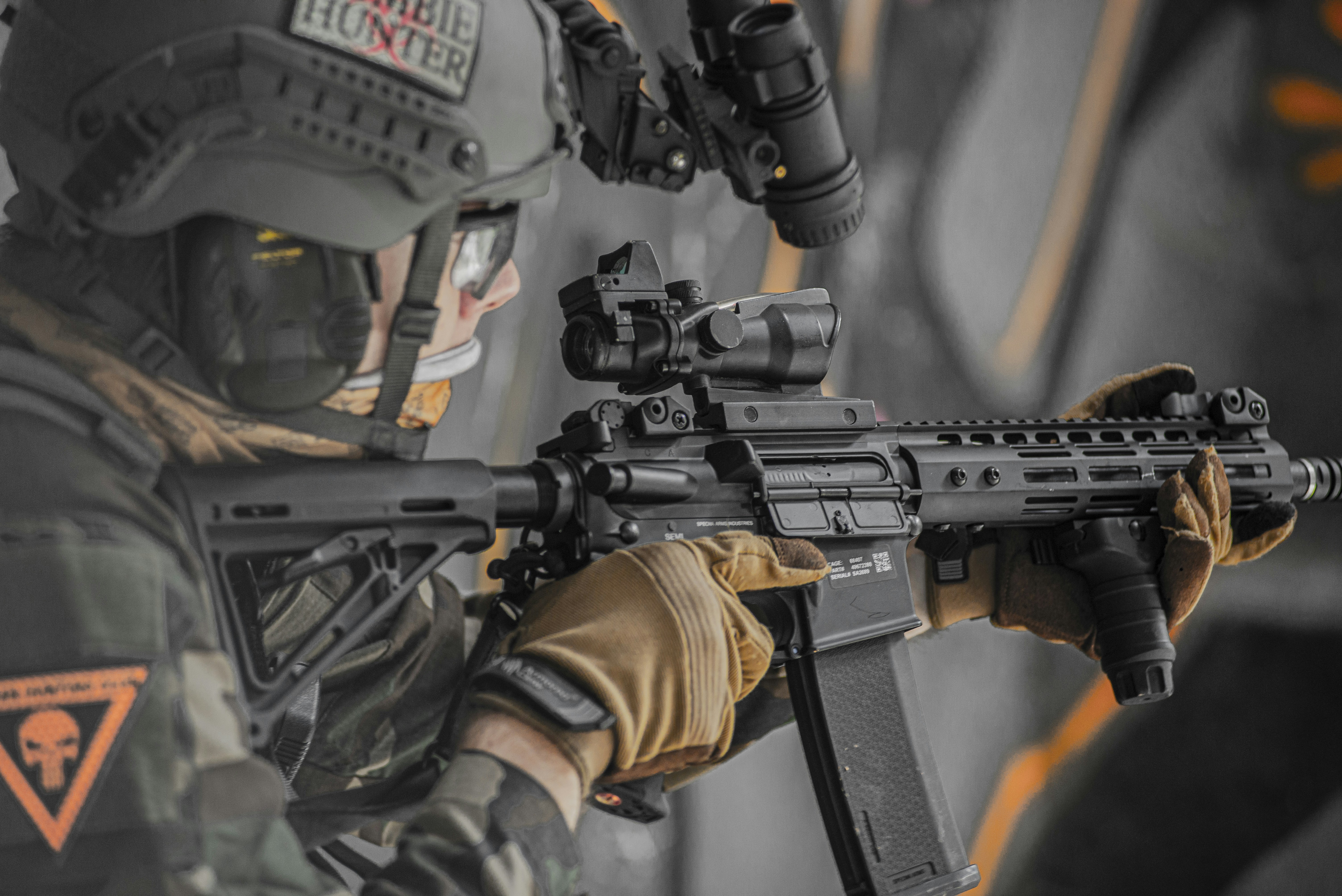
Veterans in Machine Learning: A Military‑to‑Civilian Pathway into AI Careers
Introduction Artificial intelligence is no longer relegated to sci‑fi films—it underpins battlefield decision‑support, fraud detection, and even supermarket logistics. The UK Government’s 2025 AI Sector Deal forecasts an additional £200 billion in GDP by 2030, with machine‑learning (ML) engineers cited as the nation’s second most in‑demand tech role (Tech Nation 2024). The Ministry of Defence’s Defence AI Strategy echoes that urgency, earmarking £1.6 billion for FY 2025–28 to embed ML into planning, logistics, and autonomous systems. If you have ever tuned a radar filter, plotted artillery trajectories, or sifted sensor data for actionable intel, you have already worked with statistical modelling—the backbone of machine learning. This guide shows UK veterans how to reframe military experience for ML roles, leverage MoD transition funding, and land high‑impact positions building the models shaping tomorrow’s defence and commercial landscapes. Quick Win: Bookmark our live board for Machine‑Learning Engineer roles to see who’s hiring today.

Rural-Remote Machine Learning Jobs: Finding Balance Beyond the Big Cities
Over the past decade, machine learning (ML) has transformed from a niche research domain into a pervasive technology underpinning everything from recommendation systems and voice assistants to financial forecasting and autonomous vehicles. Historically, the UK’s major tech hubs—particularly London—have been magnets for top ML talent and corporate headquarters. However, remote work has become mainstream, and many ML professionals are realising they can excel in their field while living far beyond the city limits. At MachineLearningJobs.co.uk, we’ve observed a growing interest in positions that allow for a rural lifestyle or a coastal environment, often reflected in search terms like “ML remote countryside” or “tech jobs by the sea.” This surge is no coincidence. Flexible work policies, better rural broadband, and the nature of machine learning tasks—much of which can be done through cloud platforms—are bringing new opportunities to those who wish to swap urban hustle for fresh air and scenic views. Whether you’re a data scientist, ML engineer, researcher, or product manager, a rural or seaside move could reinvigorate your work-life balance. In this article, we’ll unpack why rural-remote ML jobs are on the rise, how you can navigate the challenges of leaving the city, and what you need to do to thrive in a machine learning career beyond the M25. If you’ve dreamt of looking up from your laptop to rolling fields or ocean waves, keep reading—your rural ML role might be closer than you think.

Quantum-Enhanced Machine Learning—Propelling AI into the Next Frontier
Machine learning (ML) has revolutionised how we interpret data, build predictive models, and create intelligent applications. From recommendation engines and self-driving cars to advanced genomics and natural language processing, ML solutions are integral to nearly every corner of modern life. However, as data complexity and model size continue to skyrocket, the computational demands placed on ML systems grow in tandem—often pushing even high-performance classical computers to their limits. In recent years, quantum computing has emerged as a tantalising solution to these challenges. Unlike traditional digital systems, quantum computers exploit quantum mechanics—superposition and entanglement—to process information in ways that defy conventional logic. As these machines mature, they promise exponential speed-ups for certain tasks, potentially reshaping how we approach AI and data-intensive challenges. What does this mean for machine learning? Enter quantum-enhanced ML, a new frontier where quantum processors and classical ML frameworks unite to accelerate model training, tackle high-dimensional data, and solve complex optimisation tasks more efficiently. In this article, we will: Unpack the current state of machine learning, highlighting key bottlenecks. Provide a concise overview of quantum computing—why it’s radical and how it differs from classical technology. Examine potential breakthroughs in quantum-enhanced ML, including real-world use cases and technical approaches. Explore the roles and skill sets that will define this quantum-AI era, with guidance on how to prepare. Discuss the roadblocks (like hardware maturity and ethical concerns) and how they might be addressed in the years to come. If you’re a machine learning engineer, data scientist, or simply an AI enthusiast fascinated by the next wave of computational innovation, read on—quantum computing could become an integral part of your future toolkit, opening up job opportunities and reimagining what ML can achieve.