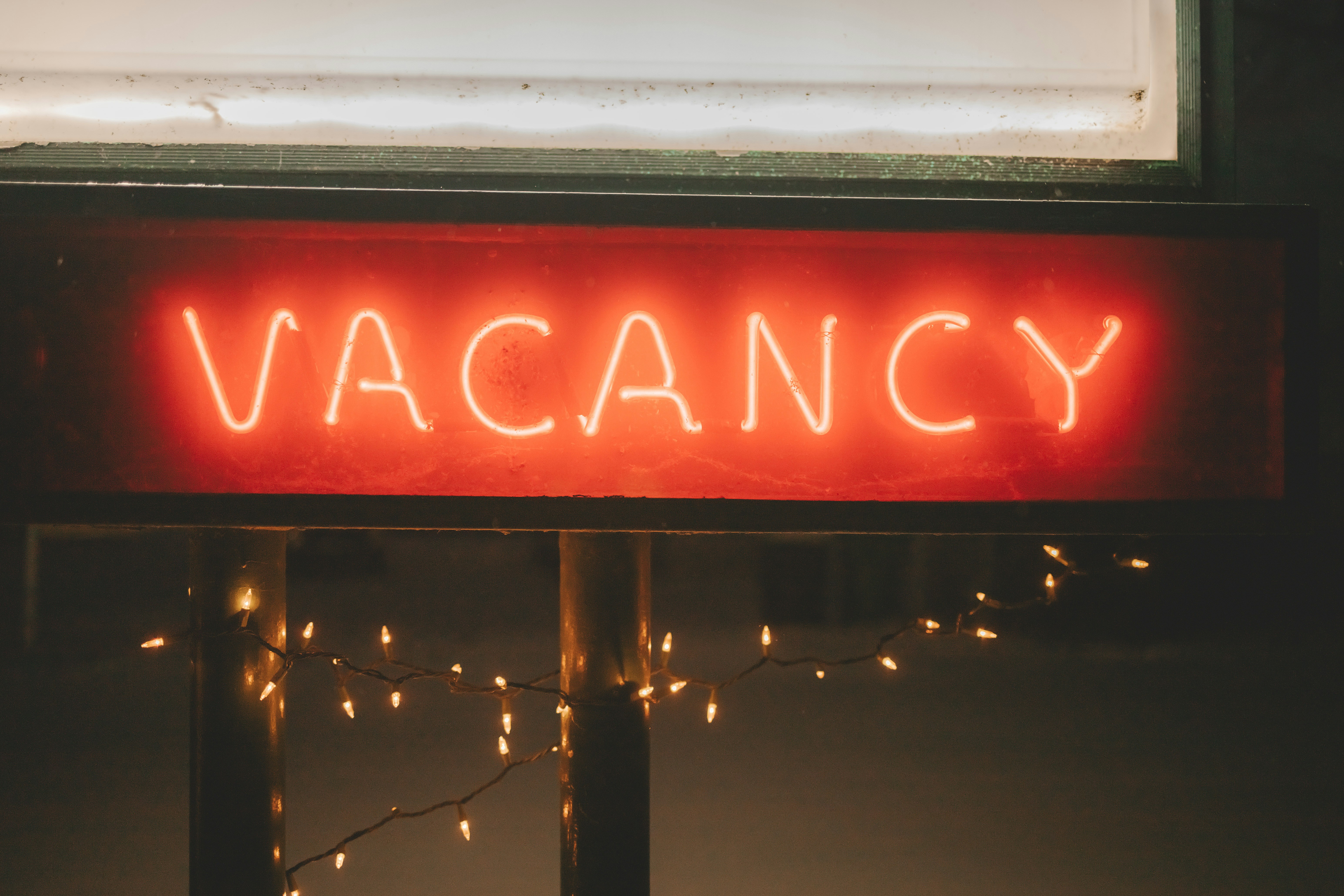
How to Hire Machine Learning Talent: A Comprehensive Guide for Recruiters
Machine Learning (ML) is no longer just a buzzword; it’s a transformative technology reshaping industries ranging from finance and healthcare to e-commerce and manufacturing. As businesses recognise the strategic value of data-driven insights and predictive modelling, the demand for machine learning professionals has skyrocketed. For recruiters, this creates both an enormous opportunity and a significant challenge: finding, attracting, and retaining the right ML talent to drive innovation and competitiveness.
In this in-depth guide, we’ll explore why the market for ML professionals is so heated, highlight the most in-demand roles and responsibilities, and offer practical tips to refine your hiring strategy. We’ll also show how www.machinelearningjobs.co.uk can help you connect with top-tier candidates in the UK. Additionally, we’ll discuss our LinkedIn community and how engaging with it can enhance your recruitment efforts.
Table of Contents
Why Demand for Machine Learning Talent Is Booming
Key Machine Learning Roles in Today’s Market
Essential Skills and Qualifications
Challenges in Recruiting ML Professionals
Best Practices for Hiring ML Specialists
Leveraging www.machinelearningjobs.co.uk
6.1 Targeted ML Talent Pool
6.2 SEO-Optimised Listings
6.3 Employer Branding
6.4 Advanced Filtering & Analytics
6.5 Dedicated Support
6.6 Join Our LinkedIn Community
Cultivating an Attractive ML Employer Brand
Retention Strategies for ML Talent
Industry Sectors Embracing Machine Learning
Future Trends in ML Recruitment
Frequently Asked Questions (FAQs)
Conclusion and Next Steps
1. Why Demand for Machine Learning Talent Is Booming
1.1 Widespread Adoption
Machine learning has grown beyond tech giants. Today, companies of all sizes—start-ups, SMEs, and global corporations—employ ML to gain insights, automate decision-making, and predict future trends. As data becomes a central asset, ML expertise is crucial to turn it into actionable knowledge.
1.2 Shifting Consumer Expectations
Customers now expect personalised experiences, real-time recommendations, and instant customer service—all powered by ML models. Businesses that fail to meet these expectations risk losing market share to data-savvy competitors.
1.3 Technological Advancements
Rapid improvements in computing power, cloud services, and open-source libraries (e.g., TensorFlow, PyTorch) lower the barrier to entry for ML. As a result, more enterprises seek talent capable of leveraging these evolving tools and frameworks.
1.4 Government and VC Funding
Governments and venture capitalists recognise ML’s potential, pouring money into AI/ML research and start-ups. This funding creates additional roles, intensifying competition for skilled developers, data scientists, and ML engineers.
2. Key Machine Learning Roles in Today’s Market
Understanding the diversity of ML roles is essential for recruiters who want to match specific projects with the right skill sets. Here are the most common ML-related positions:
Machine Learning Engineer
Develops and deploys ML models, ensuring scalability and reliability in production.
Strong emphasis on software engineering best practices and performance tuning.
Data Scientist
Focuses on extracting insights from data, using statistical analysis and ML.
Collaborates with business stakeholders to clarify data-driven hypotheses and experiments.
ML Researcher
Explores cutting-edge techniques in deep learning, reinforcement learning, or natural language processing.
Often works in R&D departments or academic-industry joint labs.
Data Engineer / MLOps Engineer
Builds and maintains the data pipelines and infrastructure needed to train ML models at scale.
Ensures ML workflows are robust, automated, and continuously monitored.
AI Product Manager
Defines product roadmaps that incorporate ML features or solutions.
Balances technical feasibility with user needs and commercial goals.
Computer Vision Specialist
Designs models for image classification, object detection, and video analytics.
Commonly employed in autonomous vehicles, medical imaging, and security.
NLP Engineer
Specialises in language models, chatbots, speech recognition, and text analytics.
Uses frameworks like Hugging Face, NLTK, or spaCy.
3. Essential Skills and Qualifications
3.1 Technical Skills
Programming Mastery: Proficiency in Python is standard in ML; C++ or Rust can be vital for performance-critical tasks.
Math & Statistics: Foundational knowledge of linear algebra, calculus, and probability is crucial for model development.
Algorithmic Expertise: Understanding algorithms (random forests, gradient boosting, neural networks) and best practices (feature engineering, hyperparameter tuning, cross-validation).
Big Data Tools: Familiarity with distributed computing systems (Spark, Hadoop) and cloud-based ML services (AWS, Azure, GCP).
Version Control & CI/CD: Ability to manage code and model changes via Git, plus automate deployments using Kubernetes or Docker.
3.2 Soft Skills
Problem-Solving: ML solutions often require iterative testing and refining of hypotheses.
Collaboration: Coordination with data engineers, product managers, and domain experts is integral.
Communication: Ability to explain complex models and outcomes to non-technical audiences.
Adaptability: ML evolves quickly. Professionals who embrace continuous learning will thrive.
4. Challenges in Recruiting ML Professionals
4.1 Shortage of Skilled Talent
Despite increasing interest, universities and bootcamps can’t meet the explosive industry demand for ML experts. Competition for seasoned professionals is intense, driving up salaries and benefits.
4.2 Rapidly Evolving Landscape
Machine learning frameworks and best practices change frequently. Recruiters face the challenge of evaluating candidates who can quickly adapt to new tools and algorithms.
4.3 High Compensation Expectations
In-demand ML roles command premium salaries, especially in tech hubs like London and Cambridge. Companies must offer competitive pay, equity, or perks to secure top candidates.
4.4 Ambiguous Role Definitions
“Machine Learning Engineer” can mean different things depending on a company’s size and focus. Clearly defining the role is crucial to attract suitable applicants.
4.5 Retention
Once onboarded, ML professionals often receive multiple external offers. Providing an environment that supports growth and innovation is key to retention.
5. Best Practices for Hiring ML Specialists
5.1 Clear Role Definition
Skills & Experience: Specify the frameworks, programming languages, and domain expertise needed.
Deliverables: Outline expected contributions—model deployment, new feature development, data pipeline optimisation, etc.
Career Path: Highlight potential for advancement within the ML team or organisation.
5.2 Robust Interview Process
Technical Screening: Coding challenges, take-home projects, or code reviews to test programming ability.
ML Challenges: Present real-world data sets or hypothetical use cases to gauge problem-solving and modelling prowess.
Culture Fit: Evaluate how candidates communicate, collaborate, and handle setbacks.
5.3 Competitive Compensation & Perks
Flexible Work: Remote or hybrid setups, especially if you’re sourcing talent outside major tech hubs.
Upskilling Opportunities: Training budgets, conference passes, and certification sponsorships to keep ML employees motivated.
Clear Incentives: Performance-based bonuses, stock options, or profit-sharing can tip the scales in your favour.
5.4 Swift Hiring Process
Time is critical—avoid drawn-out hiring cycles. Efficient scheduling, prompt feedback, and decisive offers help you secure top talent before they look elsewhere.
6. Leveraging www.machinelearningjobs.co.uk
6.1 Targeted ML Talent Pool
www.machinelearningjobs.co.uk is dedicated to connecting machine learning professionals with UK-based opportunities. By advertising here, you tap into an audience already primed to seek or continue a career in ML.
6.2 SEO-Optimised Listings
Our platform is built to rank highly for keywords like “machine learning jobs UK” and “ML engineer roles.” This ensures that top-tier candidates discover your postings quickly and easily.
6.3 Employer Branding
Show off your organisation’s culture, innovative projects, and mission. Provide insights into your tech stack, R&D initiatives, and what sets your company apart in the ML space.
6.4 Advanced Filtering & Analytics
Easily manage incoming applications using our platform’s filtering tools. Gain insights into click-through rates, application conversions, and candidate source channels, empowering you to refine your recruitment strategy.
6.5 Dedicated Support
Our team understands the complexities of machine learning. We help recruiters craft compelling job adverts, ensure optimal visibility, and offer tips for screening and interviewing candidates with niche expertise.
6.6 Join Our LinkedIn Community
In addition to posting jobs, you can expand your reach by engaging with our LinkedIn page: Machine Learning Jobs. This community is a space for:
Networking with ML professionals and fellow recruiters.
Sharing thought leadership, company updates, and hiring needs.
Staying informed on the latest machine learning trends through discussions and posts.
By participating on LinkedIn, you’ll strengthen your employer brand, stay abreast of industry news, and directly connect with potential candidates in a more personal, interactive setting.
7. Cultivating an Attractive ML Employer Brand
7.1 Innovation-Focused Culture
ML experts thrive on experimentation and real-world impact. Spotlight your organisation’s R&D initiatives or partnerships with universities and research labs.
7.2 Opportunity for Ownership
Professionals often seek autonomy in designing and deploying ML models. Advertise the chance to own entire projects or initiatives, encouraging accountability and creativity.
7.3 Continuous Learning
Offer internal workshops, hackathons, or knowledge-sharing sessions. Show that you genuinely invest in employees’ long-term skill development.
7.4 Public Recognition
Encourage team members to present at conferences or publish articles. Their public accomplishments elevate both individual careers and your brand reputation.
8. Retention Strategies for ML Talent
8.1 Growth & Learning
Mentorship Programmes: Pair junior ML hires with senior mentors to facilitate rapid skill growth.
Innovation Days: Allocate time for side projects, enabling employees to experiment with new ideas.
8.2 Collaborative Environment
Cross-Functional Teams: Pair ML experts with product managers, data engineers, and domain specialists.
Regular Feedback: Structured reviews that recognise achievements and clarify objectives.
8.3 Competitive Benefits
Health & Wellness: Private health insurance, mental health support, and fitness subsidies can stand out.
Financial Incentives: Performance bonuses, salary reviews, and equity options keep employees motivated.
8.4 Flexibility & Work-Life Balance
Allow remote or hybrid work arrangements and flexible hours where possible. This fosters trust and can reduce turnover in a competitive job market.
9. Industry Sectors Embracing Machine Learning
Retail & E-commerce
Recommendation engines, demand forecasting, and personalised marketing.
Finance & Banking
Fraud detection, algorithmic trading, credit risk assessment, and chatbot services.
Healthcare & Pharma
Diagnostics (medical imaging), drug discovery, patient risk profiling, and genomics.
Manufacturing & Supply Chain
Predictive maintenance, quality control, routing, and inventory optimisation.
Marketing & Advertising
Customer segmentation, sentiment analysis, and budget allocation optimisation.
Autonomous Vehicles & Robotics
Path planning, perception (computer vision, LIDAR processing), and motion control.
10. Future Trends in ML Recruitment
10.1 MLOps & Automation
As ML models move from prototype to production, MLOps roles will multiply. Recruiters should anticipate growing demand for engineers who can standardise deployment, monitoring, and maintenance of models.
10.2 Responsible AI & Ethics
Consumers and regulators alike are scrutinising ML models for bias and transparency. Expect dedicated roles focusing on ethical AI, fairness, and explainability.
10.3 Edge ML
With the rise of IoT and edge devices, more ML inference is happening locally rather than in the cloud. Roles requiring embedded systems knowledge and performance optimisation will see more interest.
10.4 Interdisciplinary Teams
ML is merging with quantum computing, 5G, AR/VR, and blockchain. AI professionals adept at navigating multiple tech domains will be highly sought after.
11. Frequently Asked Questions (FAQs)
Q1: How competitive are ML salaries in the UK?
Salaries vary by location and seniority, but mid-level ML engineers often command £50,000–£70,000, with senior positions potentially exceeding £100,000.
Q2: Do all ML roles require a PhD?
Not necessarily. Many roles accept candidates with Bachelor’s or Master’s degrees in Computer Science, Statistics, or a related field, as long as they can demonstrate strong ML fundamentals and practical experience.
Q3: Which programming languages are most sought after?
Python is the most common, with frameworks like TensorFlow or PyTorch. C++ or Rust may be favoured in performance-critical tasks or for certain library integrations.
Q4: How can we assess cultural fit for ML candidates?
Introduce group problem-solving exercises or pair-coding sessions during interviews. Evaluate how candidates communicate, handle feedback, and solve issues collaboratively.
Q5: What about remote work for ML professionals?
Remote and hybrid setups are increasingly common. However, certain roles requiring specialised hardware (e.g., GPU clusters) or secure data environments may require on-site presence.
Q6: How do we justify the ROI of an ML hire?
Show tangible metrics—cost reductions, improvements in forecast accuracy, faster product launches, or revenue gains—linking them back to ML projects.
12. Conclusion and Next Steps
As machine learning gains momentum, recruiting the right professionals is vital for sustainable innovation. By offering competitive compensation, clear career paths, and a culture of experimentation, you’ll attract the talent you need. However, this process becomes significantly more efficient when you tap into a specialised recruitment platform tailored to the ML field.
Accelerate Your Hiring with machinelearningjobs.co.uk
Advertise Open Roles: Post your vacancies on www.machinelearningjobs.co.uk to access a community of ML-savvy professionals.
Enhance Employer Branding: Detail your innovative projects, company culture, and growth opportunities to stand out in a crowded market.
Network on LinkedIn: Join our Machine Learning Jobs page for deeper engagement—share thought leadership, build brand presence, and connect with potential hires.
Optimise the Recruitment Process: Leverage our platform’s advanced filters, analytics, and support resources to streamline your candidate search.
By following these steps and investing in the right hiring strategy, you’ll be better positioned to source, onboard, and retain top-tier machine learning talent, ensuring your organisation can stay ahead in today’s data-driven world.
Ready to recruit the ML professionals who’ll drive your business forward? Advertise on www.machinelearningjobs.co.uk today and start building the high-performing ML team you need! Contact sales@futuretechjobs.co.uk to find out more.